A novel immune-related long noncoding RNA (lncRNA) pair model to predict the prognosis of triple-negative breast cancer
Highlight box
Key findings
• Our study constructed a risk assessment model by immune-related long noncoding RNA (irlncRNA) pairs regardless of expression levels, which contributed to predicting the efficacy of immunotherapy in triple-negative breast cancer (TNBC).
What is known and what is new?
• Previous studies have revealed that TNBC is critically related to the expression of programmed cell death ligand 1 (PD-L1) in the tumor microenvironment.
• We investigated the correlation between the risk model and tumor-infiltrating immune cells and immune checkpoints. Finally, we identified USP30-AS1 through the StarBase and Multi Experiment Matrix databases, predicted the potential target genes of USP30-AS1, and then discovered that these target genes were closely associated with immune responses.
What is the implication, and what should change now?
• Our study constructed a risk assessment model by irlncRNA pairs regardless of expression levels, which contributed to predicting the efficacy of immunotherapy in TNBC. Furthermore, the lncRNA USP30-AS1 in the model was positively correlated with the expression of PD-L1 and provided a potential therapeutic target for TNBC.
Introduction
Breast cancer (BC) is the most commonly diagnosed cancer in females worldwide and is classified into different molecular subtypes, including luminal A, luminal B, human epidermal growth factor receptor-2 (HER-2) overexpression and triple-negative breast cancer (TNBC) (1,2). TNBC is characterized by a lack of estrogen receptor (ER), progesterone receptor (PR), and HER2 expression, representing approximately 15–20% of BC cases (3,4). At present, comprehensive mode therapy combining local therapy (surgery, radiotherapy) with systemic therapy (endocrine therapy, chemotherapy, etc.) is a relatively mature treatment method for BC (5,6). However, chemotherapy promotes cancer heterogeneity of tumor cells, which could result in chemoresistance and cancer development (4,5,7). TNBC is characterized by significant biological heterogeneity. Despite its advances in neoadjuvant and adjuvant therapies, overall TNBC is associated with a higher risk of relapse and disease progression and poorer outcomes (8).
The development of immunotherapeutic drugs has revolutionized the field of clinical oncology. Immune checkpoint inhibitors (ICIs) are currently available to induce durable immune responses and potential long-term benefits in different tumor types, including lung cancer, hepatocellular carcinoma, melanoma, and renal cell carcinoma (9-12). However, BC, as a “cold” cancer, is considered poorly immunogenic and immunotherapy is not a priority option (13). There is growing evidence that TNBC exhibits the strongest immunogenicity in BC (14). Compared to other subtypes, TNBC has been shown to have a higher proportion of tumor infiltrating lymphocytes (TILs) (15), a relatively high tumor mutational burden (TMB) (16) and PD-L1 expression (17). PD-L1 expression has been found in approximately 20% of TNBCs, and several clinical trials have shown that antibodies against the programmed cell death protein 1/programmed cell death ligand 1 (PD-1/PD-L1) pathway could induce immune responses and improve clinical outcomes in TNBC patients (2,18,19). Thus, immunotherapy has emerged as a promising option for the treatment of TNBC and has encouraged the development of additional immunologic agents for the treatment of TNBC patients. However, not all TNBC patients are sensitive to immunotherapy. Given the heterogeneity of TNBC, it is imperative to explore potential mechanisms for regulating the efficacy of immunotherapy in TNBC patients.
Long noncoding RNAs (lncRNAs) play a prominent role in carcinogenesis, such as regulating gene expression, cell differentiation, aggressiveness of cancer cells and their ability to metastasize (20-22). LncRNAs can be transcribed from both protein-coding and noncoding regions of DNA (23). Several lncRNAs have been reported to influence the carcinogenic process and have particular applications in the prediction and diagnosis of different cancers (24). Ma et al. observed that a metabolism-related lncRNA signature was identified that can predict the recurrence-free survival (RFS) of BC patients, and a prognostic nomogram was established that helps guide the individualized treatment of patients at different risks (25). Li et al. observed that four-lncRNA immune prognostic signatures were prognostic biomarkers and could be possible therapeutic targets in TNBC (26).
Immune system disorders play a critical role in the development of various types of cancers (27,28). LncRNAs play crucial roles in the regulation of the immune system. Over the past few years, lncRNAs have emerged as key players in epigenetic regulation in the inhibitory tumor microenvironment (TME) because of their potential role in regulating tumor immunity by directly regulating genes involved in immune activation or suppression (29,30). For example, lncRNA KCNQ1OT1 secreted by tumor cell-derived exosomes regulates PD-L1 ubiquitination via miR-30a-5p/USP22 to promote immune escape in colorectal cancer (31). However, the role of immune-related lncRNAs (irlncRNAs) in regulating malignant progression and the efficacy of immunotherapy in TNBC has rarely been investigated. Exploring the potential mechanism by which lncRNAs regulate PD-L1 expression in TNBC patients is of great significance to identify novel therapeutic targets for TNBC.
In the current study, we identified differentially expressed irlncRNAs (DEirlncRNAs) in TNBC samples according to public transcriptome profiling data and corresponding clinical data of TNBC patients. Then, we utilized a novel modelling method to construct a risk assessment model, which applied lncRNA pairs and did not require precise expression levels. We further investigated the correlation between the risk model and the tumor immune microenvironment. Finally, we predicted the target genes and performed functional annotation to explore the potential mechanisms. We present this article in accordance with the TRIPOD reporting checklist (available at https://tcr.amegroups.com/article/view/10.21037/tcr-23-1975/rc).
Methods
Gene expression profiles and clinical data
The independent data included in this study were downloaded from a public database. The data of 146 TNBC samples and corresponding clinical information were obtained from The Cancer Genome Atlas (TCGA, https://portal.gdc.cancer.gov/) database. In addition, the clinical characteristics of all TNBC patients are shown in Table 1. This study was conducted in accordance with the Declaration of Helsinki (as revised in 2013).
Table 1
Variables | TCGA cohort (N=146) | |
---|---|---|
N | % | |
Status | ||
Alive | 125 | 85.62 |
Dead | 21 | 14.38 |
Age (years) | ||
≥55 | 73 | 50.00 |
<55 | 73 | 50.00 |
AJCC-T | ||
T1 | 37 | 25.34 |
T2 | 92 | 63.01 |
T3 | 13 | 8.90 |
T4 | 4 | 2.74 |
AJCC-N | ||
N0 | 96 | 65.75 |
N1 | 31 | 21.23 |
N2 | 11 | 7.53 |
N3 | 8 | 5.48 |
AJCC-M | ||
M0 | 124 | 84.93 |
M1 | 1 | 0.68 |
MX | 21 | 14.38 |
Stage | ||
Stage I | 26 | 17.81 |
Stage II | 95 | 65.07 |
Stage III | 21 | 14.38 |
Stage IV | 1 | 0.68 |
Unknown | 3 | 2.05 |
Postoperative adjuvant chemotherapy | ||
Yes | 24 | 16.44 |
No | 1 | 0.68 |
NA | 121 | 82.88 |
Neoadjuvant therapy | ||
Yes | 1 | 0.68 |
No | 145 | 99.32 |
TCGA, The Cancer Genome Atlas; AJCC, American Joint Committee on Cancer.
Identification of differentially expressed irlncRNAs (DEirlncRNAs)
Gene transfer format (GTF) files were retrieved from Ensembl (http://asia.ensembl.org) for annotation. A list of identified immune-related genes was obtained from the ImmPort database (http://www.immport.org) and was used to distinguish irlncRNAs by coexpression analysis. Correlation analysis was performed between immune-related genes and lncRNAs. LncRNAs with immune gene correlation coefficients greater than 0.4 and P values less than 0.001 were selected as irlncRNAs. The “Limma” package was used for differentially expressed analysis among irlncRNAs to identify DEirlncRNAs. False discovery rate (FDR) <0.05 and |log2 fold change (FC)| >1 were set as the standard to select DEirlncRNAs for further analysis.
Pairing DEirlncRNAs
The matching process of DEirlncRNA pairs was as follows: one lncRNA pair included two genes (A and B); if the expression level of A was larger than that of B, the score was 1; otherwise, the score was 0. Next, DEirlncRNA pairs with constant values (0 or 1) were removed from all individual datasets included in the meta-dataset, and the remaining DEirlncRNA pairs were considered candidate DEirlncRNA pairs. Additionally, only samples with a 0.2–0.8 pair ratio, defined as the total pair value, were included.
Construction of a prognostic risk model
Univariate Cox regression analysis was performed to identify prognostic irlncRNA pairs. Least absolute shrinkage and selection operator (LASSO) regression analysis was performed with 10-fold cross validation and a P value of 0.01. Then, multivariate Cox regression analysis was utilized to calculate the coefficients (βi) of selected lncRNA pairs. A risk assessment model was constructed using βi and lncRNA expression levels to calculate the risk score of each patient. The risk score was calculated as follows: risk score = ∑βi * Expi. The TNBC patients in the TCGA cohort were stratified into two categories, namely low-risk and high-risk, based on the risk score calculated using the median value. The 2-, 3-, and 5-year ROC curves were plotted to estimate the predictive efficacy of the model. The R packages used in these steps included “survival”, “survminer”, “glmnet”, and “survivalROC”.
Evaluation of the constructed risk model
To verify the predictive value of the cut-off point, Kaplan-Meier analysis was performed to compare the difference in survival in the high- or low-risk groups. The Chi-square test was conducted to investigate the association between the model and clinicopathologic characteristics, and a band diagram was plotted to show the results. Univariate and multivariate Cox regression analyses between the risk score and clinicopathological characteristics were performed to determine whether the ability of the model to predict overall survival (OS) was independent. Box diagrams show the differences in the risk score among different groups of these clinicopathological characteristics by the Wilcoxon signed-rank test. The R packages used in these steps are “survival”, “survminer”, “ggpubr”, and “ComplexHeatmap”.
Investigation of tumor-infiltrating immune cells and immune checkpoint molecules
We further investigated the association between the risk and immune status using XCELL, TIMER, QUANTISEQ, MCPCOUNTER, EPIC, CIBERSORT-ABS, and CIBERSORT. The results were shown in a lollipop diagram generated by the R “ggplot2” package with P<0.05. The correlation between the risk score and the expression levels of immune checkpoint-related genes was exhibited in violin plots using the “ggpubr” package.
Correlation between the risk model and drug sensitivity
We calculated the half-maximal inhibitory concentration (IC50) of commonly administered chemotherapeutic drugs for TNBC in the TCGA database. The differences in the IC50 of imatinib, erlotinib, bexarotene, methotrexate and camptothecin between the high- and low-risk groups were compared using the R packages “pRRophetic” and “ggplot2”.
Target gene prediction and gene enrichment analysis
We utilized the StarBase and Multi Experiment Matrix (MEM) databases to identify potential lncRNAs in the three lncRNA pairs, and USP30-AS1 was selected. We performed coexpression analysis to predict the potential target genes of USP30-AS1 and calculated Spearman’s rank correlation coefficients. Gene Ontology (GO) and Kyoto Encyclopedia of Genes and Genomes (KEGG) pathway analyses were performed for functional annotation. “Pathview” was used to pathway-based data integration and visualization (32,33).
Statistical analysis
The Chi-square test was used to compare clinical variables. Kaplan-Meier analysis was performed to compare the difference in survival between different patient groups. The correlation was determined by Spearman correlation analysis. Survival status was analysed by Cox regression analysis. P<0.05 was considered statistically significant.
Results
Identification of DEirlncRNAs
We downloaded the transcriptome profiling data of 146 cases of TNBC patients in the TCGA dataset. The expression matrix of immune-related mRNA was obtained from the ImmPort database and was used to identify irlncRNAs by coexpression analysis. The patients were divided into a high-expression group and a low-expression group based on the median cut-off value of PD-L1 expression. A total of 58 DEirlncRNAs were identified (Figure 1A), among which 52 irlncRNAs were upregulated in the high-PD-L1 group, and 6 irlncRNAs (AC007991.2, BCL2L1-AS1, DTNB-AS1, LINC00582, LINC01781, LINC02446) were upregulated in the low-PD-L1 group (|log2FC| >1, FDR <0.05) (Figure 1B). These results indicated that irlncRNAs may be potential regulators of PD-L1 expression in TNBC patients.
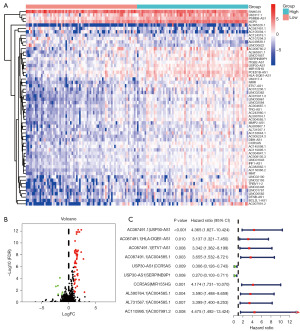
Construction of DEirlncRNA pairs and a risk assessment model
We established 1,145 DEirlncRNA pairs and performed univariate Cox regression analysis. Ten DEirlncRNA pairs with prognostic significance were identified (Figure 1C). LASSO and multivariate Cox regression analyses were applied to identify three lncRNA pairs (Table 2) and establish a risk assessment model based on the transcriptome profiling data of the above DEirlncRNAs (Figure 2A,2B). The optimal cut-off value of the 5-year ROC curve was identified as 1.058 (Figure 2C). Time-dependent ROC curve analysis was applied to illustrate the sensitivity and specificity of the prediction model, with the area under the curve (AUC) reaching 0.680 at 2 years, 0.795 at 3 years, and 0.795 at 5 years (Figure 2D). Figure 2E displayed three lncRNA pairs.
Table 2
DEirlncRNAs | Coef | HR | HR.95 L | HR.95 H | P value |
---|---|---|---|---|---|
USP30-AS1|SERPINB9P1 | −0.91874 | 0.399023 | 0.150387 | 1.058732 | 0.064989 |
AL731567.1|AC004585.1 | 1.145169 | 3.142973 | 1.265462 | 7.806065 | 0.013617 |
AC110995.1|AC007991.2 | 1.436283 | 4.205038 | 1.383095 | 12.78462 | 0.011354 |
LncRNA, long noncoding RNA; DEirlncRNAs, differentially expressed immune-related lncRNAs; Coef, coefficient; HR, hazard ratio; 95L, 95% lower; 95 H, 95% higher.
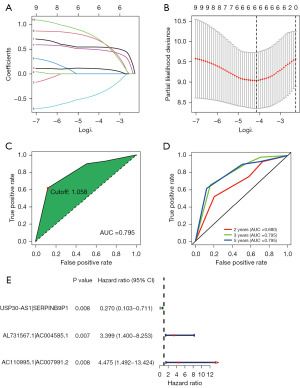
Evaluation of independent prognostic value and correlation between clinical factors and the risk model
To further verify the prognostic ability of the risk model, we calculated the risk scores of the 140 patients with TNBC in the TCGA cohort. The patients were divided into a high-risk and a low-risk group based on the identified cut-off point, of which 55 cases were in the high-risk group, and 91 cases were in the low-risk group. The Kaplan-Meier survival curve indicated that patients in the high-risk group were more likely to exhibit a worse prognosis than those in the low-risk group (P=0.029) (Figure 3A). Univariate and multivariate Cox regression analyses of the risk model were performed to determine whether the prognostic ability of the model was independent. The results of univariate Cox regression analysis showed that clinical stage [hazard ratio (HR) =11.268, 95% CI: 4.680–27.127, P<0.001], T stage (HR =3.413, 95% CI: 1.839–6.332, P<0.001), N stage (HR =3.249, 95% CI: 2.097–5.033, P<0.001), and risk score (HR =2.031, 95% CI: 1.255–3.287, P=0.004) were independent predictive factors (Figure 3B). In multivariate Cox regression analysis, clinical stage (HR =13.055, 95% CI: 2.413–70.631, P=0.003) and risk score (HR =2.518, 95% CI: 1.381–4.592, P=0.003) were still independent prognostic predictors (Figure 3C). A Chi-square test was performed to illustrate the relationship between the risk score of TNBC and clinicopathological characteristics (Figure 3D). We further investigated the correlation between age (Figure 3E), T stage (Figure 3F) and risk by the Wilcoxon signed-rank test. The risk score increased as age and T stage increased. These results showed that age and T stage were significantly related to the risk score.
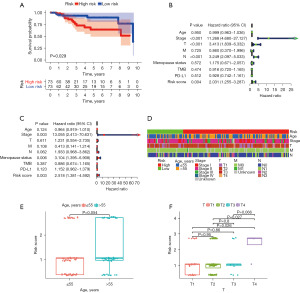
The risk model is highly correlated with tumor-infiltrating immune cells and immune checkpoint molecules
Given that irlncRNAs were identified based on the coexpression of immune genes, we investigated whether the prediction model was related to the tumor immune microenvironment. We evaluated the immune infiltration status among the samples using XCELL, TIMER, QUANTISEQ, MCPCOUNTER, EPIC, CIBERSORT-ABS, and CIBERSORT. A detailed Spearman correlation analysis was performed to study the relationship between the risk score and immune infiltrated cells. The correlation coefficients are shown in a lollipop diagram (Figure 4A) and listed in Table S1. The high-risk group was positively correlated with natural killer (NK) cells, regulatory T cells (Tregs), and M0 macrophages and negatively correlated with other immune infiltrating cells, which indicated that patients in the high-risk group may be insensitive to immunotherapy. Since checkpoint blockade therapy is extensively used in the clinical treatment of BC, we further investigated the relationship between the risk model and the expression levels of immune checkpoint molecules in the TCGA database. The results of violin plots revealed that high risk scores were positively associated with low expression of PD-1, PD-L1, PD-L2, CTLA4, TIM3, and IDO1 (P<0.05) (Figure 4B).
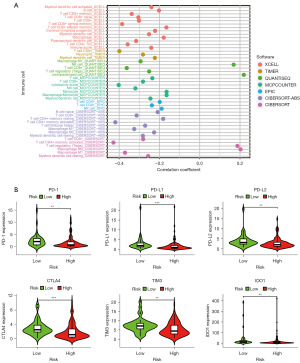
The risk model may act as a potential predictor of chemotherapeutics
To evaluate the risk model in the clinic for TNBC treatment, we identified the relationship between the risk and the efficacy of commonly administered chemotherapeutic drugs in the TCGA database. The inhibitory concentration (IC50) of chemotherapeutic drugs, including imatinib, erlotinib, bexarotene, methotrexate, and camptothecin, was calculated. The results showed that a high-risk score was related to a lower IC50 of imatinib (P=0.043), erlotinib (P=0.04), and bexarotene (P=0.095) (Figure 5A) and a higher IC50 of methotrexate (P=0.0073) and camptothecin (P=0.039) (Figure 5B), indicating that the risk model may act as a potential predictor of chemotherapeutic drug sensitivity.
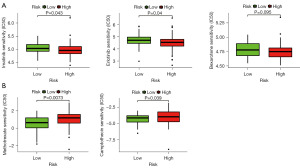
Target gene prediction and functional analysis of USP30-AS1
Among the selected DEirlncRNAs, USP30-AS1 was identified through StarBase, the MEM database and the expression matrix of irlncRNAs. We predicted the potential target genes of USP30-AS1 in the risk model and identified 941 target genes (correlation coefficients ≥0.4, P<0.05), the top 100 of which are shown in Table S2. The genes encoding PD-1 (PDCD1) and PD-L1 (CD274) were included. Spearman’s rank correlation coefficient revealed that the expression of PD-L1 (Figure 6A) and PD-1 (Figure 6B) had a significant positive correlation with the expression of USP30-AS1 (P<0.001). To identify the potential biological functions and pathways of target genes of USP30-AS1, we further performed GO analysis (Figure 6C) and KEGG pathway analysis (Figure 6D). Based on GO analysis, the target genes were significantly correlated with cytokine binding, receptor binding, cytokine activity, and several immune-related biological processes. The results of the KEGG pathway analysis revealed that the most relevant pathway for target genes was the cytokine-cytokine receptor interaction. We further generated a KEGG view of the cytokine-cytokine receptor interaction (Figure 7) and found that most genes in this pathway were positively regulated by USP30-AS1. It can be assumed that USP30-AS1 may play an important role in the immune responses of TNBC.
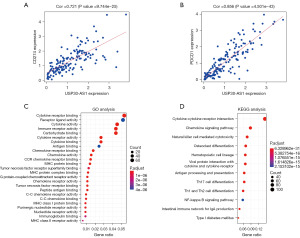
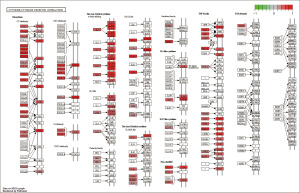
Discussion
Key findings, comparison with similar researches and explanations of findings
Previous studies have revealed that TNBC is critically related to the expression of PD-L1 in the TME (15,17). Furthermore, accumulating data have shown that PD-1/PD-L1 inhibitors are able to induce durable clinical responses in some TNBC patients (34). The KEYNOTE-355 trial suggested that pembrolizumab (an anti-PD-1 antibody)-chemotherapy showed a significant and clinically meaningful improvement in progression-free survival (PFS) versus placebo-chemotherapy among patients with metastatic TNBC (mTNBC) (35). The IMpassion130 trial revealed that atezolizumab (an anti-PD-L1 antibody) plus nab-paclitaxel significantly improved PFS and median OS in patients with mTNBC, especially for PD-L1-positive patients (36). However, the IMpassion 131 trial revealed that the combination of atezolizumab and paclitaxel did not improve PFS nor OS compared with paclitaxel alone, suggesting that the specific mechanisms of anti-PD-1/PD-L1 immunotherapies for mTNBC still need to be further explored (36,37).
In the present study, we used the cyclic single pairing method (38) and 0 or 1 matrix to verify the signatures of lncRNA pairs to predict the prognosis of TNBC. Therefore, only pairs with high or low expression need to be detected without examining the specific expression value of each lncRNA. Ten pairs with prognostic values were identified by univariate Cox regression analysis. Some lncRNAs of the ten pairs have been reported to be critically involved in tumorigenesis, such as USP30-AS1 (39-41), HLA-DQB1-AS1 (42,43), and MIR155HG (44-46). Based on the LASSO regression analysis, we constructed a risk assessment model composed of three DEirlncRNA pairs (USP30-AS1|SERPINB9P1, AL731567.1|AC004585.1, AC110995.1|AC007991.2). We further plotted ROC curves for 2, 3 and 5 years, and the model exhibited remarkable prognostic validity. The patients were divided into a high-risk and a low-risk group according to the identified cut-off point. The results of the survival analysis showed that the survival probability of the high-risk group was lower than that of the low-risk group. The results of univariate and multivariate Cox proportional hazard regression analyses showed that clinical stage and risk score were independent predictive factors for TNBC. In addition, age and T stage were significantly related to the risk score. The risk score increased as age and T stage increased. The highest increase in the risk score was found in patients with the T4 stage, which was much higher compared to patients with the T1-T3 stage. The results of the T1, T2 and T3 stages exhibited no significant difference, which may result from the limited samples of patients with low stages.
Immune checkpoint molecules have been identified as key modulators of the immune response, and their expression is closely related to the level of tumor-infiltrating immune cells, response to immunotherapy, and survival of patients (47). We evaluated the immune infiltration status among the samples using XCELL, TIMER, QUANTISEQ, MCPCOUNTER, EPIC, CIBERSORT-ABS, and CIBERSORT. We further investigated the relationship between the risk score and ICI-related biomarkers in the TCGA database. The results revealed that low risk scores were positively related to high expression of PD-1, PD-L1, PD-L2, CTLA4, TIM3, and IDO1 (P<0.05). Despite progress in understanding the underlying tumor biology, clinical outcomes for TNBC unfortunately remain poor, and chemotherapy is still the mainstay of treatment for TNBC (48-51). Thus, we evaluated the association of risk values with the IC50 of some commonly used chemotherapy agents. We further found that this risk model may be beneficial in helping physicians choose more effective chemotherapy agents.
We identified the lncRNA USP30-AS1 based on the StarBase and MEM databases. USP30-AS1 is an antisense lncRNA that plays a critical role in regulating gene expression at the replication, transcription, and translation levels (52). The function of antisense lncRNAs is not related to the position of encoding genes but to the coexpressed protein-encoding genes. We further predicted potential target genes of USP30-AS1 and discovered that genes encoding PD-1 (PDCD1) and PD-L1 (CD274) were also included, and the expression levels of PD-1 and PD-L1 were positively correlated with USP30-AS1 expression. The results indicated that USP30-AS1 may be involved in the regulation of PD-1 expression. Furthermore, the predicted target genes were used to perform GO analysis and KEGG pathway analysis. A KEGG pathway, cytokine-cytokine receptor interaction, was significantly enriched by the predicted target genes. In addition, most genes in this pathway were positively regulated by USP30-AS1, suggesting that USP30-AS1 was closely associated with the tumor immune response. Therefore, we speculate that USP30-AS1 may serve as a potential target molecule to affect the efficacy of immunotherapy, and the potential mechanism is that it activates the transcription and upregulates the expression of PD-L1 to promote the immune escape of patients with TNBC.
Limitations
However, our study has some limitations. First, our immune-related prognostic features of lncRNA pairs were developed through retrospective studies. Thus, prospective cohort studies are needed to further validate our results. Second, although functional annotation has revealed that lncRNA USP30-AS1 can regulate the expression of PD-L1, in vivo and in vitro experiments are further needed to explore the specific mechanisms.
Conclusions
In conclusion, our study constructed a risk assessment model by immune-related lncRNA pairs regardless of expression levels, which contributed to predicting the efficacy of immunotherapy in TNBC. Furthermore, the lncRNA USP30-AS1 in the model was positively correlated with the expression of PD-L1 and provided a potential therapeutic target for TNBC.
Acknowledgments
This work was supported by National Key Clinical Specialties Construction Program.
Funding: None.
Footnote
Reporting Checklist: The authors have completed the TRIPOD reporting checklist. Available at https://tcr.amegroups.com/article/view/10.21037/tcr-23-1975/rc
Peer Review File: Available at https://tcr.amegroups.com/article/view/10.21037/tcr-23-1975/prf
Conflicts of Interest: All authors have completed the ICMJE uniform disclosure form (available at https://tcr.amegroups.com/article/view/10.21037/tcr-23-1975/coif). The authors have no conflicts of interest to declare.
Ethical Statement: The authors are accountable for all aspects of the work in ensuring that questions related to the accuracy or integrity of any part of the work are appropriately investigated and resolved. This study was conducted in accordance with the Declaration of Helsinki (as revised in 2013).
Open Access Statement: This is an Open Access article distributed in accordance with the Creative Commons Attribution-NonCommercial-NoDerivs 4.0 International License (CC BY-NC-ND 4.0), which permits the non-commercial replication and distribution of the article with the strict proviso that no changes or edits are made and the original work is properly cited (including links to both the formal publication through the relevant DOI and the license). See: https://creativecommons.org/licenses/by-nc-nd/4.0/.
References
- Chen Z, Chen Z, Xu S, et al. LncRNA SOX2-OT/miR-30d-5p/PDK1 Regulates PD-L1 Checkpoint Through the mTOR Signaling Pathway to Promote Non-small Cell Lung Cancer Progression and Immune Escape. Front Genet 2021;12:674856. [Crossref] [PubMed]
- Yi H, Li Y, Tan Y, et al. Immune Checkpoint Inhibition for Triple-Negative Breast Cancer: Current Landscape and Future Perspectives. Front Oncol 2021;11:648139. [Crossref] [PubMed]
- Zhu Y, Zhu X, Tang C, et al. Progress and challenges of immunotherapy in triple-negative breast cancer. Biochim Biophys Acta Rev Cancer 2021;1876:188593. [Crossref] [PubMed]
- Damaskos C, Garmpis N, Garmpi A, et al. Investigational Drug Treatments for Triple-Negative Breast Cancer. J Pers Med 2021;11:652. [Crossref] [PubMed]
- Huang P, Ouyang DJ, Chang S, et al. Chemotherapy-driven increases in the CDKN1A/PTN/PTPRZ1 axis promote chemoresistance by activating the NF-κB pathway in breast cancer cells. Cell Commun Signal 2018;16:92. [Crossref] [PubMed]
- Gobbini E, Ezzalfani M, Dieras V, et al. Time trends of overall survival among metastatic breast cancer patients in the real-life ESME cohort. Eur J Cancer 2018;96:17-24. [Crossref] [PubMed]
- Belli C, Duso BA, Ferraro E, et al. Homologous recombination deficiency in triple negative breast cancer. Breast 2019;45:15-21. [Crossref] [PubMed]
- Abdou Y, Goudarzi A, Yu JX, et al. Immunotherapy in triple negative breast cancer: beyond checkpoint inhibitors. NPJ Breast Cancer 2022;8:121. [Crossref] [PubMed]
- Makiguchi T, Tanaka H, Kamata K, et al. Immune-mediated thrombocytopenia induced with durvalumab after chemoradiotherapy in a non-small cell lung cancer patient: A case report. Thorac Cancer 2021;12:2811-4. [Crossref] [PubMed]
- Reschke R, Gussek P, Boldt A, et al. Distinct Immune Signatures Indicative of Treatment Response and Immune-Related Adverse Events in Melanoma Patients under Immune Checkpoint Inhibitor Therapy. Int J Mol Sci 2021;22:8017. [Crossref] [PubMed]
- Hiraoka A, Kumada T, Tada T, et al. Efficacy of lenvatinib for unresectable hepatocellular carcinoma based on background liver disease etiology: multi-center retrospective study. Sci Rep 2021;11:16663. [Crossref] [PubMed]
- Marchetti A, Rosellini M, Rizzo A, et al. An up-to-date evaluation of cabozantinib for the treatment of renal cell carcinoma. Expert Opin Pharmacother 2021;22:2323-36. [Crossref] [PubMed]
- Chen C, Li A, Sun P, et al. Efficiently restoring the tumoricidal immunity against resistant malignancies via an immune nanomodulator. J Control Release 2020;324:574-85. [Crossref] [PubMed]
- Liu Z, Li M, Jiang Z, et al. A Comprehensive Immunologic Portrait of Triple-Negative Breast Cancer. Transl Oncol 2018;11:311-29. [Crossref] [PubMed]
- García-Teijido P, Cabal ML, Fernández IP, et al. Tumor-Infiltrating Lymphocytes in Triple Negative Breast Cancer: The Future of Immune Targeting. Clin Med Insights Oncol 2016;10:31-9. [Crossref] [PubMed]
- Thomas A, Routh ED, Pullikuth A, et al. Tumor mutational burden is a determinant of immune-mediated survival in breast cancer. Oncoimmunology 2018;7:e1490854. [Crossref] [PubMed]
- Meng Y, Liang H, Hu J, et al. PD-L1 Expression Correlates With Tumor Infiltrating Lymphocytes And Response To Neoadjuvant Chemotherapy In Cervical Cancer. J Cancer 2018;9:2938-45. [Crossref] [PubMed]
- Steiner M, Tan AR. The evolving role of immune checkpoint inhibitors in the treatment of triple-negative breast cancer. Clin Adv Hematol Oncol 2021;19:305-15. [PubMed]
- Mittendorf EA, Philips AV, Meric-Bernstam F, et al. PD-L1 expression in triple-negative breast cancer. Cancer Immunol Res 2014;2:361-70. [Crossref] [PubMed]
- Ghafouri-Fard S, Azimi T, Taheri M. Cervical carcinoma high expressed 1 (CCHE1): An oncogenic lncRNA in diverse neoplasms. Biomed Pharmacother 2021;142:112003. [Crossref] [PubMed]
- Ghafouri-Fard S, Khoshbakht T, Taheri M, et al. A review on the role of PCAT6 lncRNA in tumorigenesis. Biomed Pharmacother 2021;142:112010. [Crossref] [PubMed]
- Xin X, Li Q, Fang J, et al. LncRNA HOTAIR: A Potential Prognostic Factor and Therapeutic Target in Human Cancers. Front Oncol 2021;11:679244. [Crossref] [PubMed]
- Dhanoa JK, Sethi RS, Verma R, et al. Long non-coding RNA: its evolutionary relics and biological implications in mammals: a review. J Anim Sci Technol 2018;60:25. [Crossref] [PubMed]
- Schmitt AM, Chang HY. Long Noncoding RNAs in Cancer Pathways. Cancer Cell 2016;29:452-63. [Crossref] [PubMed]
- Ma JY, Liu SH, Chen J, et al. Metabolism-related long non-coding RNAs (lncRNAs) as potential biomarkers for predicting risk of recurrence in breast cancer patients. Bioengineered 2021;12:3726-36. [Crossref] [PubMed]
- Li YX, Wang SM, Li CQ. Four-lncRNA immune prognostic signature for triple-negative breast cancer Running title: Immune lncRNAs predict prognosis of TNBC. Math Biosci Eng 2021;18:3939-56. [Crossref] [PubMed]
- Chen YG, Satpathy AT, Chang HY. Gene regulation in the immune system by long noncoding RNAs. Nat Immunol 2017;18:962-72. [Crossref] [PubMed]
- Pi YN, Qi WC, Xia BR, et al. Long Non-Coding RNAs in the Tumor Immune Microenvironment: Biological Properties and Therapeutic Potential. Front Immunol 2021;12:697083. [Crossref] [PubMed]
- Hur K, Kim SH, Kim JM. Potential Implications of Long Noncoding RNAs in Autoimmune Diseases. Immune Netw 2019;19:e4. [Crossref] [PubMed]
- Vishnubalaji R, Shaath H, Elango R, et al. Noncoding RNAs as potential mediators of resistance to cancer immunotherapy. Semin Cancer Biol 2020;65:65-79. [Crossref] [PubMed]
- Xian D, Niu L, Zeng J, et al. LncRNA KCNQ1OT1 Secreted by Tumor Cell-Derived Exosomes Mediates Immune Escape in Colorectal Cancer by Regulating PD-L1 Ubiquitination via MiR-30a-5p/USP22. Front Cell Dev Biol 2021;9:653808. [Crossref] [PubMed]
- Luo W, Brouwer C. Pathview: an R/Bioconductor package for pathway-based data integration and visualization. Bioinformatics 2013;29:1830-1. [Crossref] [PubMed]
- Luo W, Pant G, Bhavnasi YK, et al. Pathview Web: user friendly pathway visualization and data integration. Nucleic Acids Res 2017;45:W501-8. [Crossref] [PubMed]
- Emens LA. Breast Cancer Immunotherapy: Facts and Hopes. Clin Cancer Res 2018;24:511-20. [Crossref] [PubMed]
- Cortes J, Cescon DW, Rugo HS, et al. Pembrolizumab plus chemotherapy versus placebo plus chemotherapy for previously untreated locally recurrent inoperable or metastatic triple-negative breast cancer (KEYNOTE-355): a randomised, placebo-controlled, double-blind, phase 3 clinical trial. Lancet 2020;396:1817-28. [Crossref] [PubMed]
- Schmid P, Rugo HS, Adams S, et al. Atezolizumab plus nab-paclitaxel as first-line treatment for unresectable, locally advanced or metastatic triple-negative breast cancer (IMpassion130): updated efficacy results from a randomised, double-blind, placebo-controlled, phase 3 trial. Lancet Oncol 2020;21:44-59. [Crossref] [PubMed]
- Miles D, Gligorov J, André F, et al. Primary results from IMpassion131, a double-blind, placebo-controlled, randomised phase III trial of first-line paclitaxel with or without atezolizumab for unresectable locally advanced/metastatic triple-negative breast cancer. Ann Oncol 2021;32:994-1004. [Crossref] [PubMed]
- Hong W, Liang L, Gu Y, et al. Immune-Related lncRNA to Construct Novel Signature and Predict the Immune Landscape of Human Hepatocellular Carcinoma. Mol Ther Nucleic Acids 2020;22:937-47. [Crossref] [PubMed]
- Chen M, Chi Y, Chen H, et al. Long non-coding RNA USP30-AS1 aggravates the malignant progression of cervical cancer by sequestering microRNA-299-3p and thereby overexpressing PTP4A1. Oncol Lett 2021;22:505. [Crossref] [PubMed]
- Wan J, Guo C, Fang H, et al. Autophagy-Related Long Non-coding RNA Is a Prognostic Indicator for Bladder Cancer. Front Oncol 2021;11:647236. [Crossref] [PubMed]
- Gao M, Wang X, Han D, et al. A Six-lncRNA Signature for Immunophenotype Prediction of Glioblastoma Multiforme. Front Genet 2021;11:604655. [Crossref] [PubMed]
- Wu L, Wen Z, Song Y, et al. A novel autophagy-related lncRNA survival model for lung adenocarcinoma. J Cell Mol Med 2021;25:5681-90. [Crossref] [PubMed]
- Jin D, Song Y, Chen Y, et al. Identification of a Seven-lncRNA Immune Risk Signature and Construction of a Predictive Nomogram for Lung Adenocarcinoma. Biomed Res Int 2020;2020:7929132. [Crossref] [PubMed]
- Shen L, Li Y, Hu G, et al. MIR155HG Knockdown Inhibited the Progression of Cervical Cancer by Binding SRSF1. Onco Targets Ther 2020;13:12043-54. [Crossref] [PubMed]
- Ren XY, Han YD, Lin Q. Long non-coding RNA MIR155HG knockdown suppresses cell proliferation, migration and invasion in NSCLC by upregulating TP53INP1 directly targeted by miR-155-3p and miR-155-5p. Eur Rev Med Pharmacol Sci 2020;24:4822-35. [PubMed]
- Zhang C, Liu H, Xu P, et al. Identification and validation of a five-lncRNA prognostic signature related to Glioma using bioinformatics analysis. BMC Cancer 2021;21:251. [Crossref] [PubMed]
- Tu L, Guan R, Yang H, et al. Assessment of the expression of the immune checkpoint molecules PD-1, CTLA4, TIM-3 and LAG-3 across different cancers in relation to treatment response, tumor-infiltrating immune cells and survival. Int J Cancer 2020;147:423-39. [Crossref] [PubMed]
- Vagia E, Mahalingam D, Cristofanilli M. The Landscape of Targeted Therapies in TNBC. Cancers (Basel) 2020;12:916. [Crossref] [PubMed]
- Kumar P, Aggarwal R. An overview of triple-negative breast cancer. Arch Gynecol Obstet 2016;293:247-69. [Crossref] [PubMed]
- Bianchini G, Balko JM, Mayer IA, et al. Triple-negative breast cancer: challenges and opportunities of a heterogeneous disease. Nat Rev Clin Oncol 2016;13:674-90. [Crossref] [PubMed]
- Kwapisz D. Pembrolizumab and atezolizumab in triple-negative breast cancer. Cancer Immunol Immunother 2021;70:607-17. [Crossref] [PubMed]
- Xu JZ, Zhang JL, Zhang WG. Antisense RNA: the new favorite in genetic research. J Zhejiang Univ Sci B 2018;19:739-49. [Crossref] [PubMed]