Identification and validation of the clinical prediction model and biomarkers based on chromatin regulators in colon cancer by integrated analysis of bulk- and single-cell RNA sequencing data
Highlight box
Key findings
• In this study, a novel colon adenocarcinoma (COAD) clinical prediction model was constructed. PKM screened by machine learning algorithms was associated with a wide range of drugs and affected prognosis in a variety of cancers.
What is known and what is new?
• Chromatin regulators is closely associated with tumor development.
• A novel COAD clinical prediction model was constructed. PKM may be the chromatin regulator that played a key role in COAD.
What is the implication, and what should change now?
• Clinical prediction model can provide clinical recommendations. Screened PKM may be associated with multiple drugs and require further study.
Introduction
Colon cancer is an adenocarcinoma that is caused by several lifestyle, nutritional, and hereditary factors (1). Colon adenocarcinoma (COAD) is the second leading reason for cancer-associated death, and its mortality rate among young people is progressively rising (2). Treatment options for colon cancer include total or partial colectomy and resection of the colon by endoscopic surgery, followed by radiotherapy or chemotherapy (3). In recent years, attempts have been made to apply the immune system to assess prognosis and treat patients with colon cancer, showing that the immunity system may be the way to overcome tumors (4-6). Due to the heterogeneity of the tumor microenvironment, researchers are studying colon cancer tumor microenvironment and immunotherapy targets through multi-omics to provide new options and directions for colon cancer treatment (7,8).
Clinical predictive modeling is intended for use in healthcare settings, where healthcare practitioners can use a patient’s clinical data to calculate the absolute risk of an event occurring. Clinicians can then use the risk information to guide care, and cancer patients can use their individualized risk to guide self-management (9). Then, although many clinical prediction models are currently developed, it is difficult for clinicians to choose a model that can be applied to their clinical work. This may be due to the poor usability and reproducibility of current clinical prediction models, the risk of bias in a small number of models, and the quality of reporting of prediction models that limits clinicians’ choices (10,11). More and more clinical prediction models are emerging, however, harmonized standards still do not seem to exist. Nonetheless, we still need clinical predictive modeling to provide options for clinical work, as it may serve as a basis for clinicians to diagnose and treat.
Chromatin regulators (CRs) are crucial upstream regulators for epigenetics and a way of elucidating primary cancer management. CRs may be divided into the following three classifications: DNA methylators, histone modifiers, and chromatin remodelers (12-14). CRs may be involved in colon carcinogenesis, metastasis, and drug resistance. Radhika Mathur et al. found that ARID1A, belonging to CRs, acts as a tumor inhibitor in the mouse colon and that invasive ARID1A-deficient adenocarcinoma resembles human colorectal cancer (15). Depletion of BMI1, a member of CRs, can reduce proliferation and result in apoptosis of epithelial and leukemic cell lines, and in murine colorectal cancer xenograft models (16-19). Epigenetic regulation is one of the key mechanisms of immune checkpoint expression in the tumor microenvironment (20). Li et al. identified DNASE1L3, an enzyme that regulates autoimmune responses to its own DNA and chromatin, as a potential neoregulator of antitumor immunity and a tumor suppressor in colon cancer (21). MTA1 affects downstream gene expression by participating in chromatin remodeling. Zhou et al. demonstrated that the regulation of tumor-associated macrophages (TAM) by MTA1 could affect the anti-tumor effects of cytotoxic T-lymphocytes (CTL) in the tumor microenvironment of colorectal cancer (22). CRs have been shown to provide recommendations for the diagnosis as well as the treatment of many diseases. Currently, researchers have found that immune-related CRs have an important role in idiopathic pulmonary fibrosis (IPF). Moreover, researchers have found that clinical prediction models constructed on the basis of immune-related CRs have excellent diagnostic performance and provide an important basis for the diagnosis, treatment and prognosis assessment of IPF patients (23). However, in colon cancer, research on CRs is still ongoing.
Now, researchers have identified functional aberrations in individual CRs in multiple cancer types. In addition, many CRs have been found to have dysregulated gene expression in different types of cancer (24). For instance, it has been found that up-regulated expression of EZH2, a lysine methyltransferase, promotes tumor cell proliferation by increasing promoter occupancy of H3K27 trimethylation in various cancers (25-27). Further, patients with mutations in DNMT3A, a DNA methyltransferase, have a poorer prognosis compared to patients without DNA methyltransferase mutations (28). However, the effect of multiple CRs synergizing on cancer remains unclear.
Because of the role of CRs in colon cancer being unclear, this paper provides a comprehensive analysis to identify the function of CRs in colon malignancy. We constructed a clinical prediction model from The Cancer Genome Atlas (TCGA) and Gene Expression Omnibus (GEO) data and analyzed the correlation of the model with the tumor microenvironment, immune infiltration, immune checkpoints, mutations, methylation, and therapy. This research aims to provide a prognostic model for clinical diagnosis and cancer treatment, as well as to screen key genes that can be used as drug targets. We present this article in accordance with the TRIPOD reporting checklist (available at https://tcr.amegroups.com/article/view/10.21037/tcr-23-1886/rc).
Methods
Data gathering and collation
The TCGA COAD (https://portal.gdc.cancer.gov/) group’s clinical features and mRNA expression were gathered from the UCSC database (https://xenobrowser.net/datapages/). Furthermore, the GEO database (https://www.ncbi.nlm.nih.gov/geo/) was queried for clinical information and mRNA expression linked with the GSE39582 (29) and GSE17537 (30,31) patient datasets. The same filtering process was performed for the TCGA and GEO cohorts, removing missing values and values that could not be evaluated. Finally, the TCGA cohort contained 375 tumor samples and 32 normal samples, the GSE39582 cohort collected 419 tumor samples, and the GSE17537 cohort had 55 tumor samples. Data from GSE132465 were used for single-cell analysis (32). There were 870 CRs obtained from a previous study by Lu et al. (24).
Gene enrichment and function analysis
Based on CRs, the “clusterProfiler” R package was employed for Gene Ontology (GO) and Kyoto Encyclopedia of Genes and Genomes (KEGG) analyses (33). The false discovery rate (FDR) threshold was set at FDR <0.05. GO functional analysis consists of three segments: biological processes, molecular functions, and cellular components.
KEGG analysis revealed pathways in which CRs may be involved.
Differentially expressed genes (DEGs) analysis
We quantified the genes utilizing the compute counts per million (CPM) function in the edgeR R program, then filtered the low-expressed genes. R package “limma” was employed to select DEGs (34). We set tumor samples with normal samples for analysis. The astringent filter of |logFC| >1 and a set P value <0.05 was applied to identify reliable DEGs.
Weighted Gene Co-Expression Network Analysis (WGCNA)
There were 3,309 DEGs included for WGCNA analysis (35) (R package “WGCNA”) (available online: https://cdn.amegroups.cn/static/public/tcr-23-1886-1.docx). We screened for genes in the top 75% of median absolute deviation (MAD) with at least MAD >0.01. An investigation of hierarchical clustering eliminated outliers from the research. The best power cutoff for the soft threshold was set at R2 =0.88 and mean connectivity =0. Based on the ideal values, weighted gene co-expression networks were formed, and all gene expressions and co-expression modules were recognized and grouped based on their similarity. The lowest number of genes in every module was adjusted to 30, and the module-merging cutoff was set at 0.25. The degree of association between genes was computed utilizing metric of topological overlap measure (TOM) (36). We calculated correlations between each module and clinical information and correlations between gene salience and gene connectivity within modules to identify vital clinical modules for subsequent analysis. Genes in the key modules were intersected with CRs to obtain key CRs that were clinically relevant and belong to DEGs.
Prognostic model construction based on the key CRs
For model creation, the least absolute shrinkage and selection operator (LASSO) Cox regression was employed (37) (R package “glmnet”). In brief, LASSO Cox regression constructed a regression model by cross-validating and selecting the best λ value while extracting the regression coefficients by TCGA data. The risk score calculation equation is: gene expression1*genecoef 1 + gene expression2*genecoef 2 +…+ gene expression N*genecoef N. The genecoef used for the risk score of the validation set GSE39582 was consistent with the TCGA data. Then, univariate and multivariate Cox regression analyses were employed to assess the prognostic significance of COAD’s independent risk variables. We used a multivariate Cox-based Nomogram model to forecast the risk and prognosis of COAD.
Hub gene screening
Using univariate Cox regression (R package “survival”) and support vector machine-recursive feature elimination (SVM-RFE) (R package “e1071”), the core genes in CRs were further screened (38). Univariate Cox regression was analyzed for genes associated with patient prognosis. SVM-RFE was better than linear discriminant analysis and the approach of mean squared error for selecting relevant features and removing superfluous variables. Employing ten-fold cross-validation, SVM-RFE was performed to pick characteristics.
Single-cell analysis
We calculated the scores of model genes in the single-cell dataset by the AddModuleScore function and showed them by violin and tsne plots (R package “Seurat”). Based on this score, we looked at the distribution of the model gene set in the tumor microenvironment, and we hoped to find the cells in which it functioned. Subsequently, we divided the cells into a high-scoring group and a low-scoring group based on the scores and observe the communication between the two groups of cells in the tumor microenvironment. Cellular communication in the tumor microenvironment was evaluated by CellChat (R package “CellChat”) (39). CellChat is a tool that predicts the primary outputs and inputs of cellular signaling, as well as how cells and signals coordinate their tasks, utilizing network analysis and pattern recognition techniques.
Immune infiltration and immune checkpoints analysis
In the Tumor Immune Estimation Resource (TIMER) database (http://timer.comp-genomics.org/), immune infiltration is evalated by the TIMER (40), xCELL (41), Cell-type Identification By Estimating Relative Subsets Of RNA Transcripts (CIBERSORT) (42), the quantification of the Tumor Immune contexture from human RNA-seq data (QUANTISEQ) (43), Microenvironment Cell Populations counter (MCP-counter) (44), and Estimate the Proportion of Immune and Cancer cells (EPIC) (45) methods. Immune checkpoint-associated genes were received from previous research (46). We employed Tumor Immune Dysfunction and Exclusion (TIDE, http://tide.dfci.harvard.edu) to anticipate patient response after immunotherapy (47).
Gene mutation and methylation analysis
The copy number variation (CNV), single nucleotide variation (SNV), and methylation analysis was performed in the Gene Set Cancer Analysis (GSCA) online analysis platform (http://bioinfo.life.hust.edu.cn/web/GSCALite/) (48). The platform only shows meaningful results. GSCALite is a web-based cancer gene set analysis platform that analyzes alterations in the DNA or RNA of cancer gene sets.
Drugs sensitivity analysis
To evaluate the model in predicting the clinical response of COAD treatment, we calculated the IC50 of all targeted agents in pRRophetic R package (49). The pRRophetic program predicts the clinical chemotherapeutic response employing gene expression and drug sensitivity datasets from cell lines in the Cancer Genome Project (CGP) (49,50). On the GSCA online analytic platform, the relationship between genes and drug sensitivity was evaluated (48).
Quantitative real-time reverse transcription-polymerase chain reaction (qRT-PCR)
We collected tissue samples for qRT-PCR from Northern Jiangsu People’s Hospital. 2X SG Fast qPCR Master Mix (High Rox, B639273, BBI, ABI) was used for the RT step to produce cDNA, which was used as a template in the qPCR step using the QuantStudio 1 Real-Time Fluorescence PCR System (QuantStudioTM 1 Plus System, ABI/Thermo Fisher, Foster, CA, USA). The primer sets are displayed in Table S1. The qRT-PCR assays were performed three times independently.
Pan-cancer analysis
We conducted a pan-cancer study of the most central gene in the Kaplan-Meier plotter database (https://kmplot.com/analysis/) (51,52). Kaplan-Meier Plotter, a commonly used site for survival analysis, is able to assess the correlation between the expression of genes in 21 tumors and patient survival, thereby identifying and validating biomarkers associated with survival.
Ethics approval
The patient participating in the study gave informed consent. The Medical Ethics Committee of Northern Jiangsu People’s Hospital approved the study on March 17th, 2021 (No. 2021ky104). This study was conducted in accordance with the Declaration of Helsinki (as revised in 2013).
Statistical analysis
R.4.1.3 and GraphPad Prism 8.0.2 were employed to accomplish data analysis and visualization. Some figures in this research were plotted using the ggplot2 R tool (53). When P<0.05, all findings were deemed statistically significant. The figures were illustrated by *P<0.05, **P<0.01, ***P<0.001, and ****P<0.0001.
Results
Gene enrichment and function analysis
After screening mRNAs in the TCGA cohort, a total of 859 CRs were finally involved in the study. GO analysis was divided into three parts: biological process analysis revealed that CRs participated in histone modification, chromatin organization, peptidyl-glutamine modification, chromatin remodeling, and other processes; SWI/SNF superfamily-type complex, histone acetyltransferase complex, ATPase complex, protein acetyltransferase complex, and other components were the primary focus of cellular element study; analysis of molecular function revealed histone binding, transcription coregulator activity, modification-dependent protein binding, transcription coactivator activity, and other roles (Figure 1A). The KEGG signaling mechanism analysis was enriched for cell cycle, Viral carcinogenesis, Thermogenesis, Transcriptional misregulation in cancer, Ubiquitin-mediated proteolysis, Wnt signaling mechanism, and other processes (Figure 1B). These findings were consistent with the role of CRs described in the preceding article.
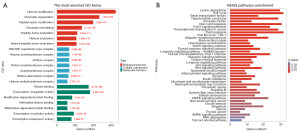
The selection of hub genes based on CRs
We obtained 3,309 DEGs after setting the |logFC| >1 and a set P value <0.05 in the TCGA cohort (available online: https://cdn.amegroups.cn/static/public/tcr-23-1886-1.docx). These DEGs were performed in the WGCNA analysis. A total of 2,481 DEGs were screened through MDA for analysis. After TOM analysis, we identified nine modules (Figure 2A). The relation heatmap between modules and clinical characteristics showed that the turquoise module (cor=0.86, P=2.8e−118) had the maximum correlation (Figure 2B). We evaluated the relation between gene significance (GS) and gene connectivity in each module based on normal traits and tumor traits separately. In normal traits, GS in the turquoise module (cor=0.47, P=2.7e−25) and brown module (cor=0.41, P=3.2e−15) were highly correlated with connectivity (Figure S1). Yellow module (cor=0.42, P=8.6e−16) and green module (cor=0.37, P=3.3e−12) also had the same characteristics in tumor traits (Figure S2). After comprehensive comparison of the correlation, we finally obtained 435 genes in the turquoise module and 332 genes in the green module for analysis. Eventually, we obtained 32 CRs for downstream analysis after intersecting the selected 767 DEGs and 859 CRs (Figure 2C).
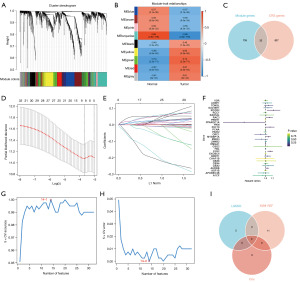
The LASSO Cox regression analysis screened eight CRs (RCOR2, PPARGC1A, PKM, RAC3, PHF19, MYBBP1A, ORC1, EYA2) for prognostic model construction (Figure 2D,2E; ST. 2). Genes were represented by lines, and the values of the vertical coordinates they pointed to were the gene coefficients calculated by LASSO Cox regression analysis (Figure 2E).The univariate Cox regression showed RCOR2 (HR: 1.12, P=0.02), PKM (HR: 1.00, P=0.01), RAC3 (HR: 1.06, P=0.03), MYBBP1A (HR: 1.04, P=0.02), EYA2 (HR: 1.07, P=0.00) had high hazard ratio and PPARGC1A (HR: 0.75, P=0.05) has low hazard ratio (Figure 2F). Fourteen CRs (MYBBP1A, EYA2, CBX8, CHAF1B, CBX4, MIER3, PCNA, RCC1, APOBEC3B, PKM, HMGA1, CBX2, PRMT1, UHRF1) was screened in SVM-RFE analysis (Figure 2G,2H). Combining the results of three machine algorithms, PKM, MYBBP1A, and EYA2 were screened (Figure 2I).
Prognostic model construction based on the LASSO Cox regression analysis
We constructed a clinical prediction model based on the results of LASSO Cox regression (Figure 3). We divided the TCGA cohort into two risk groups based on the median value of the risk score, and the GEO cohorts were treated the same way (Table S2). Both the TCGA cohort (P=0.0004) and the GSE39582 cohort (P=0.0076) showed that the low-risk cohort had better OS (Figures 3A-3C,4A-4C). Nevertheless, the GSE17537 cohort showed no significance in OS versus subgroup, despite the poorer prognosis in the high-risk group (Figure S3). The high-risk cohort had bad status and survival time, higher pathological staging, higher TNM staging, and more females than the low-risk cohort in both cohorts. However, differences existed in age: the low-risk cohort in the TCGA cohort had more patients over 60 years of age, while the GSE39582 cohort has the opposite results (Figures 3D,4D; Table 1). The ROC curves examined the anticipated impact of the risk score for OS. The area under the curve (AUC) reached 0.645 (1 year), 0.659 (3 years), and 0.659 (5 years) in the TCGA cohort (Figure 3E), and 0.61 (1 year), 0.561 (3 years), and 0.595 (5 years) in the GSE39582 cohort (Figure 4E). GSE17537 also showed the same performance (Figure S3). The univariate Cox regression illustrated that age, T, N, M, and risk score were risk factors for the prognosis of COAD in the TCGA and GSE39582 cohorts (Figures 3F,4F). The multivariate Cox regression revealed that age, T, and risk score in the TCGA cohort and age, T, N, M, and risk score in the GSE39582 cohort were risk factors (Figures 3G,4G). Stage was a protected factor in the GSE39582 cohort through the multivariate Cox regression, which was the object to the result in the univariate Cox regression (Figure 4F-4G). In addition, We used the nomogram model constructed according to multivariate Cox regression to anticipate the prognostic risk of COAD (Figures 3H,4H). Calibration curves showed a high degree of accuracy in predicting actual observations at 1, 3, and 5 years (Figures 3I,4I; Figure S3).
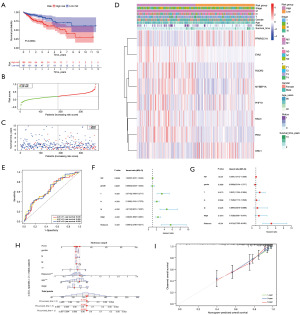
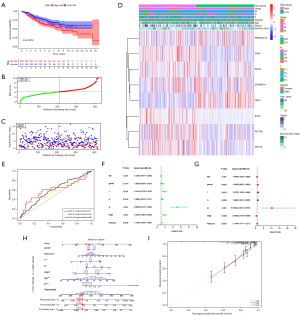
Table 1
Clinical characteristic | TCGA cohort | GSE39582 cohort | |||
---|---|---|---|---|---|
High risk group | Low risk group | High risk group | Low risk group | ||
T | |||||
1 | 3 | 6 | 4 | 5 | |
2 | 28 | 40 | 13 | 20 | |
3 | 144 | 139 | 136 | 144 | |
4 | 28 | 19 | 56 | 41 | |
N | |||||
0 | 114 | 133 | 97 | 127 | |
1 | 50 | 41 | 57 | 53 | |
2 | 39 | 30 | 50 | 29 | |
3 | 5 | 1 | |||
M | |||||
0 | 165 | 175 | 178 | 188 | |
1 | 38 | 29 | 31 | 22 | |
Stage | |||||
1 | 28 | 40 | 9 | 13 | |
2 | 80 | 89 | 83 | 107 | |
3 | 57 | 46 | 86 | 68 | |
4 | 38 | 29 | 31 | 22 | |
Age (years) | |||||
>60 | 142 | 147 | 63 | 61 | |
≤60 | 61 | 57 | 146 | 149 | |
Gender | |||||
Female | 96 | 93 | 99 | 98 | |
Male | 107 | 111 | 110 | 112 |
TCGA, The Cancer Genome Atlas; T, tumor; N, node; M, metastasis.
The performance of the eight genes in the predictive model based on single-cell analysis
We chose GSE132465 for single-cell analysis (32) (Figure 5A). We calculated CRscore based on the eight genes in the predictive model through the AddMouduleScore function (R package “Seurat”). We found that CRscore was enriched in Epithelial cells and Myeloids (Figure 5B,5C). Cellchat analysis was performed to infer intercellular communication, and signaling pathways participated in communication (39). We divided Epithelial cells and Myeloids into two cohorts based on their respective median values of CRscore. The number of interactions analysis revealed little difference in the interactions between the CRscore high Epithelial cells group and the CRscore low Epithelial cells group with other cells. However, the interaction weights between the CRscore high Epithelial cells group with Myeloids, T cells, and B cells was stronger than the CRscore low Epithelial cells group. The number and weights of interactions showed that the CRscore high Myeloid cells group had more interactions with T cells and B cells than the CRscore low Myeloid cells group (Figure 5D-5E). We found that GRN-SORT1 pathway had different performance in the CRscore high Epithelial cells group with Stromal cells and B cells in the tumor environment (Figure 5F). We finally analyzed the prognostic model genes in tumors and found that PKM has higher expression than other genes in six cells (Figure 5G).
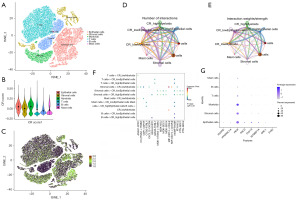
Immune infiltration
Depending on the six methods in the TIMER database, B cells and CD4+ memory T cells were enriched in the reduced-risk cohort. Based on the CIBERSORT algorithm, T cells follicular helper, Macrophage M2, Myeloid dendritic cell resting, Mast cell activated enriched in the low-risk cohort and T cell regulatory (Tregs), NK cell resting, Macrophage M0, Mast cell resting elevated in the high-risk cohort. T cell CD4+ naïve, T cell CD4+ central memory, T cell CD4+ effector memory, T cell NK, T cell CD4+ Th1, and stroma score had raised levels in the high-risk cohort. In contrast, T cell CD8+ naïve, T cell CD8+, common lymphoid progenitor, granulocyte-monocyte progenitor, T cell gamma delta, and T cell CD4+ Th2 were raised in the low-risk cohort according to the XCLL. Neutrophil was more elevated in the reduced-risk cohort depending on XCLL and QUANTISEQ methods. The endothelial cell was elevated in the high-risk cohort depending on XCLL and EPIC methods. In the EPIC, T cell CD8+ had raised infiltration levels in the reduced-risk cohort, and NK cell was higher in the high-risk cohort. Cancer-associated fibroblasts (CAFs) infiltrated more in the high-risk group according to EPIC and MCPCOUNTER (Figure 6). Combining the results of these six methods, CAFs and Endothelial were highly infiltrated in the high-risk cohort. At the same time, B cells, Neutrophils, and T cell CD8+ had high levels in the low-risk cohort.
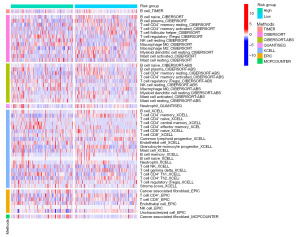
Immune checkpoints analysis and TIDE analysis
Given the significance of immune checkpoint inhibitor immunotherapies, we examined the connection between immune checkpoints and risk groups and module genes. We found significant differences in multiple immune checkpoint genes (BTLA, ICOS, CD40LG, CD48, CD28, CD200R1, ADORA2A, CD276, LGALS9, CD160, VTCN1, HHLA2, TNFSF18, BTNL2, CD70, TNFSF9, TNFRSF8, C10orf54, TNFRSF4, TNFRSF18, CD44) between high-risk and low-risk groups (Figure 7A). The expression of BTLA, ICOS, CD40LG, CD48, CD28, CD200R1, CD160, HHLA2, BTNL2, TNFSF18, and CD44 was elevated in the low-risk cohort and others were elevated in the high-risk cohort. PKM was the most critical gene related to many immune checkpoint genes (LAG3, CD276, PDCD1, TNFSF9, TNFRSF18, CD274) (Figure 7B). We used TIDE to anticipate the proportion of therapeutic responses in various risk groups. The results revealed that the individuals in the high-risk cohort received fewer immunotherapy responses, indicating a suboptimal outcome of immunotherapy. The individuals in the low-risk cohort received more responses, although P>0.05 (Figure 7C). We also found that lower-risk scores can get an immunotherapy response (Figure 7D).
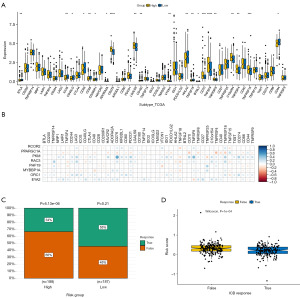
The CNV, SNV, and methylation analysis
Because CRs were closely related to CNV, SNV, and methylation, we performed CNV, SNV, and methylation analysis. Heterozygous amplification of module genes was present in COAD except for PPARGC1A, MYBBP1A, and PKM. Except for the heterozygous deletion of SLC25A15 and EYA2 in COAD, all other genes had heterozygous deletions (Figure 8A,8B). SLC25A15, MYBBP1A, PHF19, PPARGC1A, RCOR2, and EYA2 were significantly correlated to their mRNA RSEM, and MYBBP1A was the most correlated gene (Figure 8C). In the mutation analysis, PPARGC1A had the highest mutation frequency (Figure 8D). The mutation rate of MYBBP1A, PARGC1A, EYA2, PHF19, and RCOR2 was more than 10% in 43 samples (Figure 8E). In the GCSC database, the methylation level of EYA2, RAC3, RCOR2, PPARGC1A was higher in colon cancer (Figure 8F). The expression of MYBBP1A, PPARGC1A, PHF19, RCOR2, RAC3, EYA2, and SLC25A15 was negatively correlated to methylation (Figure 8G).
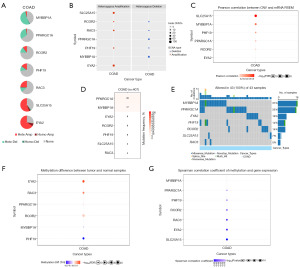
Drug sensitivity analysis
We tried to find drugs matching risk groups based on the CGP database (54). We analyzed all drugs and selected 73 drugs that had significance in the CGP database based on the R package “pRRophetic”. We found that the low-risk group was more frequently associated with more substances in comparison to the high-risk group. Eventually, we chose 12 commonly used drugs of 73 drugs. Interestingly, afatinib, AKT inhibitor VIII, epothilone B, and gemcitabine had higher sensitivity in the high-risk group, especially AKT inhibitor VIII (Figure 9A-9D). Dasatinib, docetaxel, erlotinib, gefitinib, pyrimethamine, pazopanib, paclitaxel, and sunitinib were more sensitive to the low-risk group (Figure 9E-9L). The relationship analysis between module genes and drug sensitivity was performed on the GSCA online analysis platform. The results showed that PKM was related to more drugs than other genes (Figure 10).
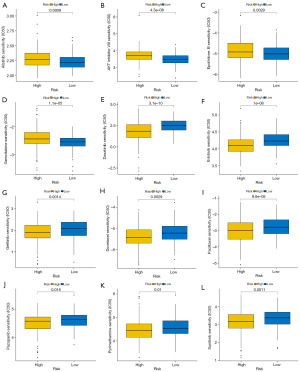
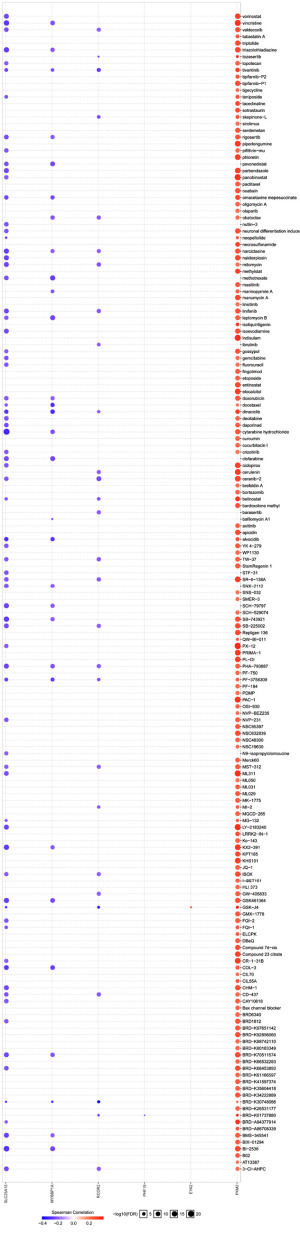
Expression validation
We explored the expression of model genes using the TCGA cohort and the GSE39582 cohort, which showed consistent results. The expression of RCOR2, PKM, RAC3, PHF19, MYBBP1A, and ORC1 was higher in cancer patients than in normal patients. In contrast, PPARGC1A and EYA2 had the opposite result (Figure 11). The effects of qRT-PCR were generally consistent with the results of the two cohorts, except for EYA2 and ORC1, which may be due to the number of samples (Figure 11).
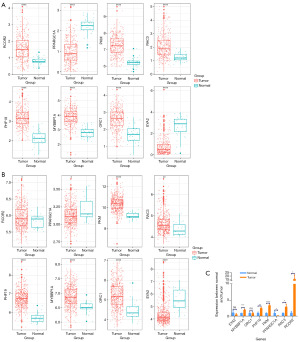
Pan-cancer analysis
Initially, we screened PKM, MYBBP1A, and EYA2 by LASSO Cox regression, SVM, and Cox regression (Figure 2I). Then, we analyzed the correlation between the predictive model genes with the tumor cells, immune checkpoints, and drug sensitivity. In contrast to other genes, PKM had a better performance. Therefore, we believed that PKM may be the most hub gene in the model. To research the effect of PKM in other cancers, we performed the pan-cancer analysis in the Kaplan-Meier plotter database. Expression analysis demonstrated that PKM was differentially expressed in all cancers except Adrenal carcinoma (Figure 12A). In the TCGA and GSE39583 cohorts, high PKM expression was associated with a bad outcome, which also can be seen in breast cancer, cervical squamous cell cancer, head-neck squamous cell cancer, liver hepatocellular cancer, lung cancer, ovarian cancer, pancreatic ductal adenocarcinoma, testicular germ cell cancer, and thymoma (P<0.05) (Figure 12B-12L). The results of the pan-cancer analysis suggested that PKM not only affected COAD but also played an essential role in other cancers, such as Liver hepatocellular carcinoma, Pancreatic ductal adenocarcinoma.
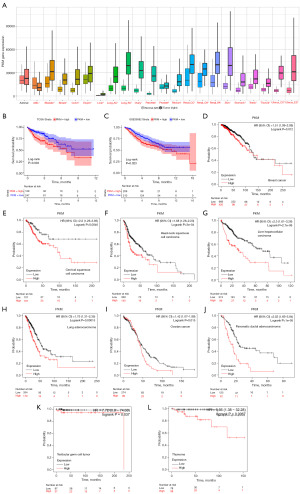
Discussion
The contribution of chromatin-regulated processes in disease and development has been intensively examined. Researchers found chromatin-organized and regulated gene mutations in more than 50% of cancers (55-57). However, comprehensive studies assessing the role of CRs in colon cancer are deficient. Therefore, we used machine learning to construct a clinical prediction model based on CRs and screened core genes. Meanwhile, we explored the model and the relationship between model genes with the tumor microenvironment, immune infiltration, immune checkpoints, CNV, SNV, methylation, and drug sensitivity. Finally, we identified the critical role of PKM in colon cancer and performed a pan-cancer analysis.
Through functional enrichment analysis of CRs, we found that their functions are mainly focused on histone modifications, chromatin regulation, transcriptome regulation, and some pathways that have been shown to impact tumor progression. This is identical to the results of previously published studies (58). The importance of chromatin in cancer has been deliberated in recent years (59-61). Therefore, research on the function of CRs is imminent.
We used WGCNA to screen for differential genes associated with clinical features and obtained 32 CRs. Eight genes were screened to construct a clinical prediction model using the LASSO Cox regression. Cox regression and SVM analysis further filtered out PKM, MYBBP1A, and EYA2 from the 32 CRs. This is our initial screening of CRs in colon cancer. Since the contribution of each gene to the model we constructed cannot be denied, in the downstream analysis, we analyzed each model gene in the hope of finding the most crucial core genes.
The model we constructed exhibits good predictive performance. To investigate the role of the model in the tumor microenvironment of colon cancer, we observed model scores, gene expression, and cellular communication utilizing single-cell data. We found that the high CRscore group communicated closely with immune cells, which may explain the poor prognosis of the high-risk group. Moreover, GRN-SORT1 pathway may play a key role. SORT1 has been promising as a tumor therapeutic target in recent years, as its expression has been increased in several types of cancers, including those of the digestive system (62-64).
Immune infiltration analysis showed that CAFs and Endothelial cells were highly infiltrated in the high-risk group. In contrast, B cells, Neutrophil, and T cell CD8+ had high levels in the low-risk group. CAFs contribute significantly to extracellular matrix maintenance, desmoplasia, angiogenesis, immunosuppression, invasion, and chemoresistance (65,66). CAFs also shape an immunosuppressive tumor microenvironment by secreting various cytokines, growth factors, chemokines, exosomes and other effector molecules that interplay with tumor-infiltrating immune cells and other immune components of the tumor immune microenvironment, enabling cancer cells to evade the immune monitoring system (67). The characteristics of CAFs may explain the low response to immunotherapy in the high-risk group. The researchers have uncovered the significance of endothelial cells in the progression of colorectal carcinogenesis, including epithelial cell proliferation, angiogenesis, and immune remodeling (68). The function of these two cell types may explain the high-risk source. The B-cell tumor promoter Bcl-3 can suppress inflammation-associated colon tumorigenesis (69). However, researchers also found that LIN28B promotes colon cancer progression by increasing B-cell lymphoma 2 expression (70). Neutrophils may also have an opposite function in cancer. Neutrophils can directly kill tumor cells in vitro and vivo (71,72), while they may also facilitate the dissemination of tumor cells by augmenting the degradation of the basement membrane (73). CD8+ T cells can release cytokines to mediate the deposition of cytotoxic particles near the target cell membrane, inducing apoptosis of tumor cells (74,75). Although the immune landscape in the low-risk group does not clearly explain its effect on cancer, the better prognosis of patients in the low-risk group suggests that tumor suppression played a significant role. In conclusion, the poor prognosis of patients in the high-risk group may be related to malignant cell infiltration.
The contribution of model genes to the model may be related to their role in cancer. RCOR2, a transcriptional repressor, plays a significant role in regulating embryonic stem cell pluripotency and neurogenesis (76). RCOR2 may affect tumor progression by altering the infiltration profile of CD8+ T cells (77). However, there are few studies on the role of RCOR2 in colon cancer. PPARGC1A, peroxisome proliferator-activated receptor γ coactivator 1A, reprogrammes tumor-specific T cells, resulting in superior intratumoral metabolic and effector function (78). PPARGC1A is associated with prognosis in patients with COAD in several studies (79,80). Overexpression of RAC3 can activate p38 and Akt kinase activity, thereby blocking the translocation of apoptosis-inducing factor-1 from the mitochondria to the nucleus, resulting in anti-apoptotic and anti-autophagic effects (81). Genome-wide analysis identifies PHF19 and TBC1D16 as oncogenic super-enhancers based on ChIP-Seq in colorectal cancer (82). MYBBP1A is a protein that binds to and stabilizes p53 and may play an important role in tumor suppression (83,84). ORC1 is essential for the viability of embryonic cells and the proliferation of intestinal epithelial cells (85). According to a previous study, ORC1 can promote the expansion of bladder cancer cells by activating Wnt/β-catenin signaling (86). EYA2, which belongs to the eyes absent family of proteins, may upregulate miR-93 expression and promote the malignant progression of breast cancer by targeting the STING signaling pathway (87).
Our study proposed that PKM may be the critical gene for CRs in colon cancer. The PKM gene can be divided into PKM1 and PKM2 by variable splicing (88). PKM1 is mainly expressed at a stable level in most tissues, while PKM2 is expressed predominantly in proliferating cells and tumor cells (89). Nuclear translocation of PKM2 or its silencing by pharmacological inhibition reduces aerobic glycolysis and tumor cell proliferation (90,91). Researchers have revealed that the HOXB-AS3 ensures the formation of lower PKM2 and suppresses glucose metabolism reprogramming in colon cancer. COAD patients with low expression of HOXB-AS3 peptide have a poorer prognosis, which PKM2 may cause (92). These findings are consistent with the results of our pan-cancer analysis.
Our study also has many limitations. We only used publicly available data for our research and did not conduct clinical trials to validate it. Furthermore, we lack basic experiments further to explore the molecular mechanisms of PKM in individual cancers.
Conclusions
In summary, we constructed an eight-gene clinical prediction model and explored the key genes in the model. Our results showed that our constructed model can be helpful to in clinical diagnosis and treatment. Finally, we found that PKM may be a beneficial target for cancer therapy, not only in colon cancer.
Acknowledgments
The authors thank Zhujiang Dai for his help with data analysis.
Funding: This work was supported by
Footnote
Reporting Checklist: The authors have completed the TRIPOD reporting checklist. Available at https://tcr.amegroups.com/article/view/10.21037/tcr-23-1886/rc
Data Sharing Statement: Available at https://tcr.amegroups.com/article/view/10.21037/tcr-23-1886/dss
Peer Review File: Available at https://tcr.amegroups.com/article/view/10.21037/tcr-23-1886/prf
Conflicts of Interest: All authors have completed the ICMJE uniform disclosure form (available at https://tcr.amegroups.com/article/view/10.21037/tcr-23-1886/coif). The authors have no conflicts of interest to declare.
Ethical Statement: The authors are accountable for all aspects of the work in ensuring that questions related to the accuracy or integrity of any part of the work are appropriately investigated and resolved. This study was conducted in accordance with the Declaration of Helsinki (as revised in 2013). The Medical Ethics Committee of Northern Jiangsu People’s Hospital approved the study on March 17th, 2021
Open Access Statement: This is an Open Access article distributed in accordance with the Creative Commons Attribution-NonCommercial-NoDerivs 4.0 International License (CC BY-NC-ND 4.0), which permits the non-commercial replication and distribution of the article with the strict proviso that no changes or edits are made and the original work is properly cited (including links to both the formal publication through the relevant DOI and the license). See: https://creativecommons.org/licenses/by-nc-nd/4.0/.
References
- Khan FA, Albalawi R, Pottoo FH. Trends in targeted delivery of nanomaterials in colon cancer diagnosis and treatment. Med Res Rev 2022;42:227-58. [Crossref] [PubMed]
- Siegel RL, Miller KD, Fuchs HE, et al. Cancer statistics, 2022. CA Cancer J Clin 2022;72:7-33. [Crossref] [PubMed]
- Janardhanam LSL, Bandi SP, Venuganti VVK. Functionalized LbL Film for Localized Delivery of STAT3 siRNA and Oxaliplatin Combination to Treat Colon Cancer. ACS Appl Mater Interfaces 2022;14:10030-46. [Crossref] [PubMed]
- Mlecnik B, Bifulco C, Bindea G, et al. Multicenter International Society for Immunotherapy of Cancer Study of the Consensus Immunoscore for the Prediction of Survival and Response to Chemotherapy in Stage III Colon Cancer. J Clin Oncol 2020;38:3638-51. [Crossref] [PubMed]
- Chalabi M, Fanchi LF, Dijkstra KK, et al. Neoadjuvant immunotherapy leads to pathological responses in MMR-proficient and MMR-deficient early-stage colon cancers. Nat Med 2020;26:566-76. [Crossref] [PubMed]
- American Association for Cancer Research. Immunotherapy Is Active in MMR-Deficient and MMR-Proficient Colon Cancer. Cancer Discov 2020;10:760. [Crossref]
- Bao X, Zhang H, Wu W, et al. Analysis of the molecular nature associated with microsatellite status in colon cancer identifies clinical implications for immunotherapy. J Immunother Cancer 2020;8:e001437. [Crossref] [PubMed]
- Zhang L, Li Z, Skrzypczynska KM, et al. Single-Cell Analyses Inform Mechanisms of Myeloid-Targeted Therapies in Colon Cancer. Cell 2020;181:442-459.e29. [Crossref] [PubMed]
- Salz T, Baxi SS, Raghunathan N, et al. Are we ready to predict late effects? A systematic review of clinically useful prediction models. Eur J Cancer 2015;51:758-66. [Crossref] [PubMed]
- Reeve K, On BI, Havla J, et al. Prognostic models for predicting clinical disease progression, worsening and activity in people with multiple sclerosis. Cochrane Database Syst Rev 2023;9:CD013606. [PubMed]
- Win AK, Macinnis RJ, Hopper JL, et al. Risk prediction models for colorectal cancer: a review. Cancer Epidemiol Biomarkers Prev 2012;21:398-410. [Crossref] [PubMed]
- Plass C, Pfister SM, Lindroth AM, et al. Mutations in regulators of the epigenome and their connections to global chromatin patterns in cancer. Nat Rev Genet 2013;14:765-80. [Crossref] [PubMed]
- Gonzalez-Perez A, Jene-Sanz A, Lopez-Bigas N. The mutational landscape of chromatin regulatory factors across 4,623 tumor samples. Genome Biol 2013;14:r106. [Crossref] [PubMed]
- Medvedeva YA, Lennartsson A, Ehsani R, et al. EpiFactors: a comprehensive database of human epigenetic factors and complexes. Database (Oxford) 2015;2015:bav067. [Crossref] [PubMed]
- Mathur R, Alver BH, San Roman AK, et al. ARID1A loss impairs enhancer-mediated gene regulation and drives colon cancer in mice. Nat Genet 2017;49:296-302. [Crossref] [PubMed]
- McGinty RK, Henrici RC, Tan S. Crystal structure of the PRC1 ubiquitylation module bound to the nucleosome. Nature 2014;514:591-6. [Crossref] [PubMed]
- Gray F, Cho HJ, Shukla S, et al. BMI1 regulates PRC1 architecture and activity through homo- and hetero-oligomerization. Nat Commun 2016;7:13343. [Crossref] [PubMed]
- Liang W, Zhu D, Cui X, et al. Knockdown BMI1 expression inhibits proliferation and invasion in human bladder cancer T24 cells. Mol Cell Biochem 2013;382:283-91. [Crossref] [PubMed]
- Kreso A, van Galen P, Pedley NM, et al. Self-renewal as a therapeutic target in human colorectal cancer. Nat Med 2014;20:29-36. [Crossref] [PubMed]
- Héninger E, Krueger TE, Lang JM. Augmenting antitumor immune responses with epigenetic modifying agents. Front Immunol 2015;6:29. [PubMed]
- Li W, Nakano H, Fan W, et al. DNASE1L3 enhances antitumor immunity and suppresses tumor progression in colon cancer. JCI Insight 2023;8:e168161. [Crossref] [PubMed]
- Zhou Y, Nan P, Li C, et al. Upregulation of MTA1 in Colon Cancer Drives A CD8(+) T Cell-Rich But Classical Macrophage-Lacking Immunosuppressive Tumor Microenvironment. Front Oncol 2022;12:825783. [Crossref] [PubMed]
- Li K, Liu P, Zhang W, et al. Bioinformatic identification and analysis of immune-related chromatin regulatory genes as potential biomarkers in idiopathic pulmonary fibrosis. Ann Transl Med 2022;10:896. [Crossref] [PubMed]
- Lu J, Xu J, Li J, et al. FACER: comprehensive molecular and functional characterization of epigenetic chromatin regulators. Nucleic Acids Res 2018;46:10019-33. [Crossref] [PubMed]
- Bachmann IM, Halvorsen OJ, Collett K, et al. EZH2 expression is associated with high proliferation rate and aggressive tumor subgroups in cutaneous melanoma and cancers of the endometrium, prostate, and breast. J Clin Oncol 2006;24:268-73. [Crossref] [PubMed]
- Visser HP, Gunster MJ, Kluin-Nelemans HC, et al. The Polycomb group protein EZH2 is upregulated in proliferating, cultured human mantle cell lymphoma. Br J Haematol 2001;112:950-8. [Crossref] [PubMed]
- Varambally S, Cao Q, Mani RS, et al. Genomic loss of microRNA-101 leads to overexpression of histone methyltransferase EZH2 in cancer. Science 2008;322:1695-9. [Crossref] [PubMed]
- Yan XJ, Xu J, Gu ZH, et al. Exome sequencing identifies somatic mutations of DNA methyltransferase gene DNMT3A in acute monocytic leukemia. Nat Genet 2011;43:309-15. [Crossref] [PubMed]
- Marisa L, de Reyniès A, Duval A, et al. Gene expression classification of colon cancer into molecular subtypes: characterization, validation, and prognostic value. PLoS Med 2013;10:e1001453. [Crossref] [PubMed]
- Chen MS, Lo YH, Chen X, et al. Growth Factor-Independent 1 Is a Tumor Suppressor Gene in Colorectal Cancer. Mol Cancer Res 2019;17:697-708. [Crossref] [PubMed]
- Smith JJ, Deane NG, Wu F, et al. Experimentally derived metastasis gene expression profile predicts recurrence and death in patients with colon cancer. Gastroenterology 2010;138:958-68. [Crossref] [PubMed]
- Lee HO, Hong Y, Etlioglu HE, et al. Lineage-dependent gene expression programs influence the immune landscape of colorectal cancer. Nat Genet 2020;52:594-603. [Crossref] [PubMed]
- Yu G, Wang LG, Han Y, et al. clusterProfiler: an R package for comparing biological themes among gene clusters. OMICS 2012;16:284-7. [Crossref] [PubMed]
- Ritchie ME, Phipson B, Wu D, et al. limma powers differential expression analyses for RNA-sequencing and microarray studies. Nucleic Acids Res 2015;43:e47. [Crossref] [PubMed]
- Langfelder P, Horvath S. WGCNA: an R package for weighted correlation network analysis. BMC Bioinformatics 2008;9:559. [Crossref] [PubMed]
- Li A, Horvath S. Network neighborhood analysis with the multi-node topological overlap measure. Bioinformatics 2007;23:222-31. [Crossref] [PubMed]
- Gui J, Li H. Penalized Cox regression analysis in the high-dimensional and low-sample size settings, with applications to microarray gene expression data. Bioinformatics 2005;21:3001-8. [Crossref] [PubMed]
- Sanz H, Valim C, Vegas E, et al. SVM-RFE: selection and visualization of the most relevant features through non-linear kernels. BMC Bioinformatics 2018;19:432. [Crossref] [PubMed]
- Jin S, Guerrero-Juarez CF, Zhang L, et al. Inference and analysis of cell-cell communication using CellChat. Nat Commun 2021;12:1088. [Crossref] [PubMed]
- Li B, Severson E, Pignon JC, et al. Comprehensive analyses of tumor immunity: implications for cancer immunotherapy. Genome Biol 2016;17:174. [Crossref] [PubMed]
- Aran D, Hu Z, Butte AJ. xCell: digitally portraying the tissue cellular heterogeneity landscape. Genome Biol 2017;18:220. [Crossref] [PubMed]
- Newman AM, Liu CL, Green MR, et al. Robust enumeration of cell subsets from tissue expression profiles. Nat Methods 2015;12:453-7. [Crossref] [PubMed]
- Finotello F, Mayer C, Plattner C, et al. Molecular and pharmacological modulators of the tumor immune contexture revealed by deconvolution of RNA-seq data. Genome Med 2019;11:34. [Crossref] [PubMed]
- Becht E, Giraldo NA, Lacroix L, et al. Estimating the population abundance of tissue-infiltrating immune and stromal cell populations using gene expression. Genome Biol 2016;17:218. [Crossref] [PubMed]
- Racle J, de Jonge K, Baumgaertner P, et al. Simultaneous enumeration of cancer and immune cell types from bulk tumor gene expression data. Elife 2017;6:e26476. [Crossref] [PubMed]
- Danilova L, Ho WJ, Zhu Q, et al. Programmed Cell Death Ligand-1 (PD-L1) and CD8 Expression Profiling Identify an Immunologic Subtype of Pancreatic Ductal Adenocarcinomas with Favorable Survival. Cancer Immunol Res 2019;7:886-95. [Crossref] [PubMed]
- Fu J, Li K, Zhang W, et al. Large-scale public data reuse to model immunotherapy response and resistance. Genome Med 2020;12:21. [Crossref] [PubMed]
- Liu CJ, Hu FF, Xia MX, et al. GSCALite: a web server for gene set cancer analysis. Bioinformatics 2018;34:3771-2. [Crossref] [PubMed]
- Geeleher P, Cox N, Huang RS. pRRophetic: an R package for prediction of clinical chemotherapeutic response from tumor gene expression levels. PLoS One 2014;9:e107468. [Crossref] [PubMed]
- Garnett MJ, Edelman EJ, Heidorn SJ, et al. Systematic identification of genomic markers of drug sensitivity in cancer cells. Nature 2012;483:570-5. [Crossref] [PubMed]
- Lánczky A, Győrffy B. Web-Based Survival Analysis Tool Tailored for Medical Research (KMplot): Development and Implementation. J Med Internet Res 2021;23:e27633. [Crossref] [PubMed]
- Bartha Á, Győrffy B. TNMplot.com: A Web Tool for the Comparison of Gene Expression in Normal, Tumor and Metastatic Tissues. Int J Mol Sci 2021;22:2622. [Crossref] [PubMed]
- Wickham H. Ggplot2: Elegant Graphics for Data Analysis: ggplot2: Elegant Graphics for Data Analysis, 2009.
- Gabor Miklos GL. The human cancer genome project--one more misstep in the war on cancer. Nat Biotechnol 2005;23:535-7. [Crossref] [PubMed]
- Beck S, Bernstein BE, Campbell RM, et al. A blueprint for an international cancer epigenome consortium. A report from the AACR Cancer Epigenome Task Force. Cancer Res 2012;72:6319-24. [Crossref] [PubMed]
- Cancer Genome Atlas Research Network. The Cancer Genome Atlas Pan-Cancer analysis project. Nat Genet 2013;45:1113-20. [Crossref] [PubMed]
- Polak P, Karlić R, Koren A, et al. Cell-of-origin chromatin organization shapes the mutational landscape of cancer. Nature 2015;518:360-4. [Crossref] [PubMed]
- Valencia AM, Kadoch C. Chromatin regulatory mechanisms and therapeutic opportunities in cancer. Nat Cell Biol 2019;21:152-61. [Crossref] [PubMed]
- Corces MR, Granja JM, Shams S, et al. The chromatin accessibility landscape of primary human cancers. Science 2018;362:eaav1898.
- Philip M, Fairchild L, Sun L, et al. Chromatin states define tumour-specific T cell dysfunction and reprogramming. Nature 2017;545:452-6. [Crossref] [PubMed]
- Zhao S, Allis CD, Wang GG. The language of chromatin modification in human cancers. Nat Rev Cancer 2021;21:413-30. [Crossref] [PubMed]
- Christou N, Blondy S, David V, et al. Neurotensin pathway in digestive cancers and clinical applications: an overview. Cell Death Dis 2020;11:1027. [Crossref] [PubMed]
- Gao F, Griffin N, Faulkner S, et al. The Membrane Protein Sortilin Can Be Targeted to Inhibit Pancreatic Cancer Cell Invasion. Am J Pathol 2020;190:1931-42. [Crossref] [PubMed]
- Yang W, Wu PF, Ma JX, et al. Sortilin promotes glioblastoma invasion and mesenchymal transition through GSK-3β/β-catenin/twist pathway. Cell Death Dis 2019;10:208. [Crossref] [PubMed]
- Ganguly D, Chandra R, Karalis J, et al. Cancer-Associated Fibroblasts: Versatile Players in the Tumor Microenvironment. Cancers (Basel) 2020;12:2652. [Crossref] [PubMed]
- Garvey CM, Lau R, Sanchez A, et al. Anti-EGFR Therapy Induces EGF Secretion by Cancer-Associated Fibroblasts to Confer Colorectal Cancer Chemoresistance. Cancers (Basel) 2020;12:1393. [Crossref] [PubMed]
- Mao X, Xu J, Wang W, et al. Crosstalk between cancer-associated fibroblasts and immune cells in the tumor microenvironment: new findings and future perspectives. Mol Cancer 2021;20:131. [Crossref] [PubMed]
- Chen WZ, Jiang JX, Yu XY, et al. Endothelial cells in colorectal cancer. World J Gastrointest Oncol 2019;11:946-56. [Crossref] [PubMed]
- Tang W, Wang H, Ha HL, et al. The B-cell tumor promoter Bcl-3 suppresses inflammation-associated colon tumorigenesis in epithelial cells. Oncogene 2016;35:6203-11. [Crossref] [PubMed]
- Yuan L, Tian J. LIN28B promotes the progression of colon cancer by increasing B-cell lymphoma 2 expression. Biomed Pharmacother 2018;103:355-61. [Crossref] [PubMed]
- Gerrard TL, Cohen DJ, Kaplan AM. Human neutrophil-mediated cytotoxicity to tumor cells. J Natl Cancer Inst 1981;66:483-8. [PubMed]
- Katano M, Torisu M. Neutrophil-mediated tumor cell destruction in cancer ascites. Cancer 1982;50:62-8. [Crossref] [PubMed]
- Uribe-Querol E, Rosales C. Neutrophils in Cancer: Two Sides of the Same Coin. J Immunol Res 2015;2015:983698. [Crossref] [PubMed]
- Trapani JA, Smyth MJ. Functional significance of the perforin/granzyme cell death pathway. Nat Rev Immunol 2002;2:735-47. [Crossref] [PubMed]
- Dunn GP, Bruce AT, Ikeda H, et al. Cancer immunoediting: from immunosurveillance to tumor escape. Nat Immunol 2002;3:991-8. [Crossref] [PubMed]
- Pei L, Zhang H, Zhang M, et al. Rcor2 Is Required for Somatic Differentiation and Represses Germline Cell Fate. Stem Cells Int 2022;2022:5283615. [Crossref] [PubMed]
- Routh ED, Pullikuth AK, Jin G, et al. Transcriptomic Features of T Cell-Barren Tumors Are Conserved Across Diverse Tumor Types. Front Immunol 2020;11:57. [Crossref] [PubMed]
- Scharping NE, Menk AV, Moreci RS, et al. The Tumor Microenvironment Represses T Cell Mitochondrial Biogenesis to Drive Intratumoral T Cell Metabolic Insufficiency and Dysfunction. Immunity 2016;45:374-88. [Crossref] [PubMed]
- Alix-Panabières C, Cayrefourcq L, Mazard T, et al. Molecular Portrait of Metastasis-Competent Circulating Tumor Cells in Colon Cancer Reveals the Crucial Role of Genes Regulating Energy Metabolism and DNA Repair. Clin Chem 2017;63:700-13. [Crossref] [PubMed]
- Sun D, Yang KS, Chen JL, et al. Identification and validation of an immune-associated RNA-binding proteins signature to predict clinical outcomes and therapeutic responses in colon cancer patients. World J Surg Oncol 2021;19:314. [Crossref] [PubMed]
- Colo GP, Rubio MF, Nojek IM, et al. The p160 nuclear receptor co-activator RAC3 exerts an anti-apoptotic role through a cytoplasmatic action. Oncogene 2008;27:2430-44. [Crossref] [PubMed]
- Li QL, Lin X, Yu YL, et al. Genome-wide profiling in colorectal cancer identifies PHF19 and TBC1D16 as oncogenic super enhancers. Nat Commun 2021;12:6407. [Crossref] [PubMed]
- Li XL, Subramanian M, Jones MF, et al. Long Noncoding RNA PURPL Suppresses Basal p53 Levels and Promotes Tumorigenicity in Colorectal Cancer. Cell Rep 2017;20:2408-23. [Crossref] [PubMed]
- Felipe-Abrio B, Carnero A. The Tumor Suppressor Roles of MYBBP1A, a Major Contributor to Metabolism Plasticity and Stemness. Cancers (Basel) 2020;12:254. [Crossref] [PubMed]
- Okano-Uchida T, Kent LN, Ouseph MM, et al. Endoreduplication of the mouse genome in the absence of ORC1. Genes Dev 2018;32:978-90. [Crossref] [PubMed]
- Chen Z, Zhou L, Wang L, et al. HBO1 promotes cell proliferation in bladder cancer via activation of Wnt/β-catenin signaling. Mol Carcinog 2018;57:12-21. [Crossref] [PubMed]
- Ren L, Guo D, Wan X, et al. EYA2 upregulates miR-93 to promote tumorigenesis of breast cancer by targeting and inhibiting the STING signaling pathway. Carcinogenesis 2021;bgab001. [PubMed]
- Noguchi T, Inoue H, Tanaka T. The M1- and M2-type isozymes of rat pyruvate kinase are produced from the same gene by alternative RNA splicing. J Biol Chem 1986;261:13807-12. [Crossref] [PubMed]
- Israelsen WJ, Vander Heiden MG. Pyruvate kinase: Function, regulation and role in cancer. Semin Cell Dev Biol 2015;43:43-51. [Crossref] [PubMed]
- Christofk HR, Vander Heiden MG, Harris MH, et al. The M2 splice isoform of pyruvate kinase is important for cancer metabolism and tumour growth. Nature 2008;452:230-3. [Crossref] [PubMed]
- Anastasiou D, Yu Y, Israelsen WJ, et al. Pyruvate kinase M2 activators promote tetramer formation and suppress tumorigenesis. Nat Chem Biol 2012;8:839-47. [Crossref] [PubMed]
- Huang JZ, Chen M, Chen D, et al. A Peptide Encoded by a Putative lncRNA HOXB-AS3 Suppresses Colon Cancer Growth. Mol Cell 2017;68:171-184.e6. [Crossref] [PubMed]