Comprehensive analysis of disulfidptosis-related lncRNAs for predicting prognosis and response of immunotherapy in pancreatic adenocarcinoma
Highlight box
Key findings
• A predictive prognosis model based on disulfidptosis-related lncRNAs was established to predict overall survival in pancreatic adenocarcinoma (PAAD).
What is known and what is new?
• It has been demonstrated that lncRNA is implicated in the genesis and development of several different tumors by way of regulating the biological behavior of tumor cells. However, the relationship between disulfidptosis-related lncRNAs and the diagnosis, prognosis, and therapeutic efficacy of immunotherapy in PAAD still needs to be further explored.
• A novel prognosis model based on disulfidptosis-related lncRNAs of PAAD has been successfully developed and verified.
What is the implication, and what should change now?
• This model could be used as a tool for predicting overall survival in PAAD, provide a new view for the clinical treatment of pancreatic cancer.
Introduction
Pancreatic cancer is a malignant tumor that is difficult to diagnose and treat, and ranks fourth in cancer-related deaths worldwide, with a 5-year survival rate of less than 9% (1). In recent years, the incidence and mortality rates of pancreatic cancer have been on the rise, and it is expected that it will overtake colorectal cancer to become the second most common cause of cancer deaths by 2040 (2). For borderline resectable pancreatic cancer (RPC), surgical treatment is undoubtedly the most effective. However, only 20% of patients have the opportunity to undergo surgery at the time of diagnosis due to the lack of early symptoms of pancreatic cancer and the presence of lymph node invasion or distant metastasis at the early stage of the disease (2). For junction RPC, neoadjuvant therapy based on FOLFIRINOX chemotherapy regimen can reduce the tumor volume or downstage, and improve the surgical resection rate of pancreatic cancer patients, but it does not significantly improve the prognosis of patients (3). Due to the “cold tumor” characteristics of pancreatic cancer tumor cells, conventional chemotherapy and radiotherapy are not effective in the treatment of locally advanced pancreatic cancer (LAPC). Immunotherapy, as a novel therapeutic strategy, has achieved encouraging results in melanoma, lung cancer, renal cancer, uroepithelial cancer and other malignant tumors. Immunotherapy for pancreatic cancer, including monoclonal antibody therapy, tumor vaccines, and chimeric antigen receptor T cell (CAR-T cell) therapy, has made some progress and demonstrated potential therapeutic effects in clinical trials. However, there are no clear biological markers to predict which patients are more likely to benefit from immunotherapy (4). Therefore, the search for a biomarker that more accurately predicts the prognosis of pancreatic cancer patients and the efficacy of immunotherapy is imminent.
Apoptosis is involved in several physiological and pathological processes in tumors. Resistance to normal death is considered to be the basis of cancer cell genesis and progression (5). In recent years, many newly discovered apoptotic cell deaths such as ferroptosis, cuproptosis, and immunogenic cell death have attracted attention (6,7). Disulfidptosis is a special mode of cell death that is different from the programmed forms of cell death that have been discovered so far, and its mechanism is that the abnormal accumulation of intracellular disulfide will cause disulfide stress, which ultimately leads to rapid cell death. A recent study has found that cells with high expression of SLC7A11, under glucose starvation, abnormally accumulate intracellular disulfide bonds such as cystine, which affects the binding between cytoskeletal proteins, leading to the collapse of the cytoskeletal protein network and rapid cell death (8). In the era of precision oncology, targeting the weakness of tumor metabolism by inducing Disulfidptosis provides new ideas in the field of cancer metabolic therapy (9). The study of Li et al. found that the disulfidptosis-related genes (DRGs), SLC7A11 and LRPPRC, play an important role in predicting the prognosis of hepatocellular carcinoma patients (10). DRGs can affect the prognosis and therapeutic effects of hepatocellular carcinoma patients by regulating the tumor microenvironment (TME), drug sensitivity and immune infiltration (11).
Long-stranded non-coding RNA (lncRNA) is a type of non-coding RNAs (ncRNAs) with a length of more than 200 nucleotides that participates in the regulation of a range of physiological and pathological processes but cannot encode proteins. It has been demonstrated that lncRNA is implicated in the genesis and development of several different tumors by way of regulating the biological behavior of tumor cells. For example, MALAT1, one of the most studied lncRNAs, is up-regulated in pancreatic cancer cells and plays a significant part in epithelial-to-mesenchymal transition (EMT)-mediated programs to promote pancreatic tumor invasion and metastasis, and its expression has been linked to patients’ chances of survival from pancreatic cancer (12). LUCAT1 is associated with non-small cell lung cancer prognosis and is involved in the proliferation, invasion and metastasis of a variety of cancer cells (13). The regulation of prostate cancer cell growth is carried out by PCGEM1. Overexpression of PCGEM1 inhibits DOX-induced apoptosis in cancer cells (14). However, the relationship between disulfidptosis-related lncRNAs (DRlncRNAs) and the diagnosis, prognosis, and therapeutic efficacy of immunotherapy in pancreatic adenocarcinoma (PAAD) still needs to be further explored.
In this research, we combined the transcription and clinical data of 178 patients with PAAD to develop a novel DRlncRNAs index (DRLI). We further elucidated the potential of the associated lncRNAs as PAAD biomarkers to predict patient prognosis, guide clinical decision-making, and specify personalized therapy. We present this article in accordance with the TRIPOD reporting checklist (available at https://tcr.amegroups.com/article/view/10.21037/tcr-23-1706/rc).
Methods
Dataset
One hundred and seventy eight PAAD patients’ RNA sequencing and related clinical details were gathered from The Cancer Genome Atlas (TCGA) (https://portal.gdc.cancer.gov/). Relevant clinical characteristics included information on age, sex, clinical grade, clinical stage and overall survival (OS). Patients lacking survival information were excluded. Subsequently, we obtained 31 DRGs from the previously published literature (10,11,15). The study was conducted in accordance with the Declaration of Helsinki (as revised in 2013).
Identification of DRlncRNAs
Pearson correlation analysis was utilized to identify DRlncRNAs. LncRNAs with P value <0.01 and an absolute Pearson correlation coefficient ≥0.4 were selected as DRlncRNAs.
Construction and validation of prognostic DRLI
We randomized PAAD patients from the TCGA database into training and validation sets. From the training sets, we screened lncRNAs having prognostic significance using univariate Cox regression analysis (P<0.05). Least absolute shrinkage and selection operator (LASSO) regression is an approach for selecting and shrinking variables in Cox proportional risk models, which improves stability and usability while reducing model complexity (16). The LASSO regression was performed in the training set by using the R package “glmnet” to identify the DRlncRNAs with the most prognostic value. Subsequently, multifactorial COX regression analysis was further used to find DRlncRNAs independently affecting prognosis to establish a DRLI.
The following is the DRLI equation:
In the equation, Coef (LncRNA) denotes the coefficient of survival-related lncRNAs, exp (LncRNA) denotes the expression of lncRNA. Depending on the median DRLI score, PAAD patients were categorized into low- and high-DRLI subgroups. Survival analysis was conducted using the R package “Survival” to verify the availability of prognostic signatures. By the R package “survivalroc”, the receiver operating characteristic (ROC) curves was plotted to verify the diagnostic value of the DRLI for 1-, 2- and 3-year survival. Subsequently, we performed analyses in the validation sets and in the whole sets to validate these results.
Independence evaluation of DRLI
The independent predictive power of the DRLI was verified by univariate and multivariate Cox regression analyses. The criterion of independence for both analyses was P<0.05. In oncology treatment, nomograms are widely used in the assessment of patient prognosis, and they facilitate clinical decision-making by condensing prediction models to quantified probabilities of an event occurring in a given circumstance for a specific patient (17). We used the R package “RMS” to establish a nomogram combining DRLI scores and clinical factors to verify the clinical viability of the DRLI. Correction curves at the 1-, 2-, and 3-year intervals were employed to validate how well their estimated and actual survival probability agreed with one another. Then, using data from the TCGA cohort, we additionally examined at the association between DRLI scores and clinical features. OS determined by DRLI score was compared in each clinical subgroup (age, sex, grade, stage) to verify the predictive value of our DRLI.
Functional enrichment analysis
To further investigate the possible mechanisms leading to the prognostic differences between the high- and low-DRLI groups, we analyzed the differentially expressed genes (DEGs, |log2FC|>1 and P<0.05) between the two subgroups. Functional enrichment analysis of DEGs was performed to explore the differences in biological processes (BPs), cellular components (CCs), molecular functions (MFs) and signaling pathways between the high- and low-DRLI groups.
Immune checkpoint analysis and immune cell infiltration analysis
By using single-sample gene set enrichment analysis (ssGSEA) based on the “gsva” R package, we evaluated distinction in immune-related functional pathways and tumor-infiltrating immune cell (TIIC) subsets between the high- and low-DRLI subgroups (18). With the help of the ESTIMATE algorithm, we examined the abundance of immune and mesenchymal stromal cells in PAAD samples to assess TME differences between the high- and low-DRLI subgroups (19). Also, immune checkpoint inhibitors’ (ICIs’) therapeutic effects might be influenced by the expression of genes associated with immunological checkpoints. By using the “ggpubr” R package, we compared the activation of immune checkpoint genes between the high- and low-DRLI subgroups.
Association between DRLI scores and tumor mutations
Patients with poorer prognosis tend to be associated with more genetic mutations. Therefore, tumor mutation burden (TMB) analysis was performed on PAAD tumor mutation data from the TCGA database in order to compare TMB values between low- and high-DRLI groups throughout the whole cohort. The R package “maftools” was used to assess mutation data. The relationship between survival and TMB status was also explored, and subgroup survival analysis was performed by combining TMB status and DRLI score.
Statistical analysis
All statistical analyses were performed with R software (4.2.2) and Perl. P<0.05 differences were statistically significant. The accuracy of the DRLI in assessing prognosis was confirmed using ROC curves and Kaplan-Meier survival analysis. Subgroups with different clinical characteristics were analyzed to fully assess the stability of the DRLI. Differences between subgroups were explored using t-tests and Wilcoxon signed rank tests.
Results
Determination of prognosis-related DRlncRNAs
We obtained the expression profiles of 31 DRGs and 16,901 lncRNAs from 178 PAAD patients from the TCGA database. Further 958 DRlncRNAs were obtained by Pearson correlation analysis (Figure 1A).
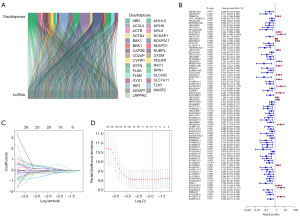
Construction of the DRLI
We randomized 178 patients with PAAD containing clinical information in the TCGA database into a training group (n=89) and a validation group (n=89). Cross-validation showed no considerable variation in clinical characteristics between the groups, demonstrating that the groups were independent of each other (P>0.05) (Table 1). In combination with clinical data, we determined the prognostic value of these DRlncRNAs by univariate Cox regression in the training subgroup. The results showed that among the 958 DRlncRNAs, 84 lncRNAs significantly linked to patient survival in PAAD (P<0.05), and a forest plot (Figure 1B) was produced to show their expression. Seven prognostic DRlncRNAs associated with OS in PAAD were identified by LASSO regression analysis in the training subgroup (Figure 1C,1D). Multifactorial COX regression analysis showed that five DRlncRNAs could independently assess the prognosis of PAAD, and the DRLI was established using these five DRlncRNAs. The DRLI was then used to assess the distribution of DRLI scores, survival status and survival time of the training set, validation set and the overall patients (Figure 2A-2C). The accuracy of the DRLI for prediction at 1, 2 and 3 years was validated by ROC curves (Figure 2D).
Table 1
Clinical feature | Type | Total | Validation | Train |
---|---|---|---|---|
Age, years | ≤65 | 94 (52.81) | 47 (52.81) | 47 (52.81) |
>65 | 84 (47.19) | 42 (47.19) | 42 (47.19) | |
Gender | Female | 80 (44.94) | 42 (47.19) | 38 (42.7) |
Male | 98 (55.06) | 47 (52.81) | 51 (57.3) | |
Grade | G1 | 31 (17.42) | 18 (20.22) | 13 (14.61) |
G2 | 95 (53.37) | 41 (46.07) | 54 (60.67) | |
G3 | 48 (26.97) | 29 (32.58) | 19 (21.35) | |
G4 | 2 (1.12) | 0 (0) | 2 (2.25) | |
GX | 2 (1.12) | 1 (1.12) | 1 (1.12) | |
Stage | I | 21 (11.8) | 9 (10.11) | 12 (13.48) |
II | 147 (82.58) | 73 (82.02) | 74 (83.15) | |
III | 3 (1.69) | 2 (2.25) | 1 (1.12) | |
IV | 4 (2.25) | 3 (3.37) | 1 (1.12) | |
Unknown | 3 (1.69) | 2 (2.25) | 1 (1.12) | |
T | T1 | 7 (3.93) | 4 (4.49) | 3 (3.37) |
T2 | 24 (13.48) | 10 (11.24) | 14 (15.73) | |
T3 | 142 (79.78) | 72 (80.9) | 70 (78.65) | |
T4 | 3 (1.69) | 2 (2.25) | 1 (1.12) | |
TX | 1 (0.56) | 0 (0) | 1 (1.12) | |
Unknown | 1 (0.56) | 1 (1.12) | 0 (0) | |
M | M0 | 80 (44.94) | 38 (42.7) | 42 (47.19) |
M1 | 4 (2.25) | 3 (3.37) | 1 (1.12) | |
MX | 94 (52.81) | 48 (53.93) | 46 (51.69) | |
N | N0 | 49 (27.53) | 21 (23.6) | 28 (31.46) |
N1 | 120 (67.42) | 63 (70.79) | 57 (64.04) | |
N1b | 4 (2.25) | 2 (2.25) | 2 (2.25) | |
NX | 4 (2.25) | 2 (2.25) | 2 (2.25) |
Data are presented as n (%).
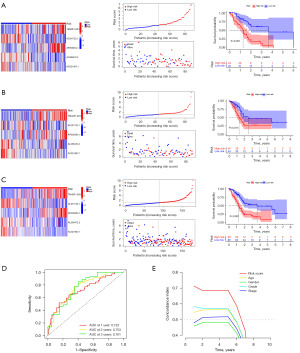
To verify the excellent predictive value of the prognostic signature associated with disulfidptosis, we compared the DRLI score with other clinical elements. The C-index of all factors of interest was calculated, and the results showed that the C-index of the DRLI score was superior to most other clinical elements (Figure 2E). These results imply that the DRlncRNAs-based index might provide a more accurate prognosis assessment for PAAD patients.
Relationship between DRLI scores and clinicopathologic features
Subgroup survival analysis validated the prognostic value of DRLI scores in patients with different clinical characteristics. According to the calculated DRLI scores, PAAD patients with different clinicopathological characteristics were categorized into low-DRLI and high-DRLI groups, respectively. Kaplan-Meier survival analyses showed that patients in the low-DRLI subgroups were significantly relevant to poorer survival outcomes except those with stages III–IV (Figure 3A-3H). The Figures 3I further shows the distribution of clinicopathologic features and DRLI scores.
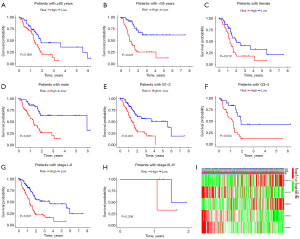
Independent prognostic analysis and the establishment of nomogram
DRLI scores were analyzed as a factor influencing the prognosis of PAAD patients in univariate and multivariate COX regression analyses along with traditional clinical parameters. The results showed that DRLI score could be used as an independent prognostic predictor [univariate hazard ratio (HR) =1.167, 95% confidence interval (CI): 1.102–1.236, P<0.001; multivariate HR =1.168, 95% CI: 1.099–1.241, P<0.001] (Figure 4A,4B). Compared to the other clinicopathologic parameters, the DRLI score’s area under the curve (AUC) value was greater (Figure 4C). Several clinical factors and DRLI scores were included in the nomogram to better predict survival outcomes in PAAD patients (Figure 4D). Subsequently, a calibration curve was plotted to investigate the accuracy of this nomogram, and the results that followed indicated that the actual values were generally consistent with the predicted values (Figure 4E). Overall, our nomogram is a useful tool to estimate a patient’s prognosis for PAAD.
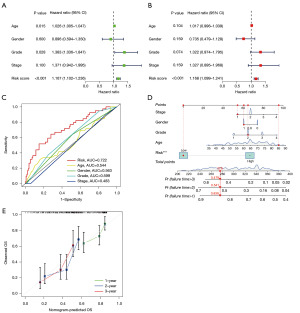
Exploration of cellular processes and signaling pathways affected by DRlncRNAs
By analyzing the functional enrichment of 897 DEGS between the high- and low-DRLI subgroups, we investigated the potential biological pathways and functions between the two subgroups. Gene Ontology (GO) functional enrichment analysis and Kyoto Encyclopedia of Genes and Genomes (KEGG) analysis point to a strong relationship between DEGs and a variety of activities and processes, including those involved in the control of chemical synaptic transmission, the cyclic adenosine monophosphate (cAMP) signaling pathway, and the mitogen-activated protein kinase (MAPK) signaling network (Figure 5A,5B).
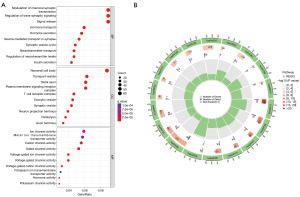
Analysis of tumor immune infiltration
We further investigated the tumor immune landscape of PAAD in the TCGA database. We explored the infiltration profiles of 16 immune cells by ssGSEA algorithm. The results are shown in Figure 6A: between the high- and low-DRLI subgroups, there were appreciable differences in the infiltration profiles of five TIICs. It is interesting to note that none of these cells were as high as those in the PAAD high-risk subgroup. The ssGSEA algorithm was subsequently applied to assess the immune-related functions between the two subgroups. The high-DRLI subgroups showed less levels of immune function (Figure 6B). The ESTIMATE algorithm revealed that the low-DRLI subgroups had higher immunity scores, stroma scores, and estimated scores (Figure 6C-6E). This suggests that the purity of tumors in the high-DRLI subgroup was higher. The above results suggest that an immunosuppressive tumor immune microenvironment exists in the high-DRLI subgroup, which may be a contributing factor to the poorer prognosis of this patient subgroup. In addition, the expression analysis of immune checkpoint genes was also negatively correlated with DRLI score (Figure 6F). In conclusion, these results provide new insights for future individualized and precise treatment of patients in different risk subgroups.
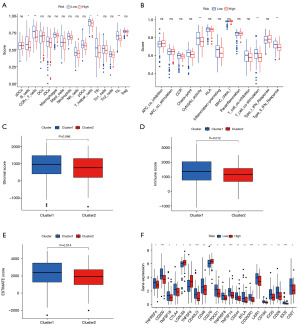
Tumor mutation analysis
We examined the TMB profiles of two subgroups and identified the top 20 mutated genes (Figure 7A). The low TMB group had better survival outcomes (Figure 7B). The combined analysis of TMB and DRLI score showed that the low DRLI + low TMB group had better survival than the other three groups (Figure 7C). These results suggest that DRLI score has better prognostic predictive ability compared to TMB.

Discussion
To date, the molecular mechanisms underlying the pathogenesis of PAAD are not fully understood. LncRNAs are crucial in the incidence and progression of PAAD according to several studies (20). PAAD-associated lncRNAs can act as oncogenic or tumor suppressor lncRNAs and serve as potential markers for assessing the survival and diagnosis of patients with PAAD (21). For example, LNC00976, a tumor suppressor lncRNA, is a good predictor of PAAD prognosis. Further studies have shown down-regulation of LINC00976 expression would result in reduced proliferation, migration potential and invasiveness of pancreatic cancer cells in vivo and in vitro (22). Upregulation of LINC00462 resulted in EMT of PAAD cells and could interact with miR-665 to increase the metastatic and invasive power of PAAD cells (23). On the contrary, LINC01111, a newly discovered lncRNA, was shown to inhibit various biological functions of PAAD cells, such as proliferation, metastasis, cell survival, and cell cycle progression in vitro experiments (24). However, studies on disulfidptosis-related lncRNAs are few, particularly in PAAD.
In this study, we included 178 patients with PAAD from the TCGA dataset. First, we identified 84 lncRNAs with prognostic significance associated with disulfidptosis by univariate Cox regression analysis. Five lncRNAs (including TM4SF1.AS1, AL031722.1, AP005264.1, AL358472.2, AC021087.1) were selected by LASSO regression and multifactorial Cox regression analysis to develop a predictive signature for estimating OS in PAAD patients. On the basis of their median DRLI scores, patients were classified into low- and high-DRLI groupings. The results of survival analysis revealed a substantial difference between the high-DRLI and low-DRLI groups in survival. ROC curves were applied to confirm the validity and dependability of the DRLI’s prediction capability. To test its validation furtherly, we repeated all analyses in validation set and entire set. We also used DRLI scores together with other clinical factors to construct nomogram plots to quantitatively predict 1-, 2-, and 3-year survival rate in individual PAAD patients, thereby providing recommendations for clinical diagnosis and treatment.
Among the five lncRNAs used to construct the model, TM4SF1.AS1 and AP005264.1 were potential risk factors, while AL031722.1, AL358472.2 and AC021087.1 were protective factors. A previous study has found that TM4SF1-AS1 contributes to tumorigenesis by inducing stress granule formation and inhibiting apoptosis (25). In hepatocellular carcinoma, TM4SF1-AS1 promotes tumor cell proliferation, migration, and invasion by enhancing TM4SF1 expression (26). M4SF1-AS1 is upregulated in gastric cancer tissues, and affects the ability of cells to proliferate, invade and transition from epithelium to mesenchyme through the PI3K-AKT signaling pathway (27). Lin et al. found that AL031722.1 may be associated with the prognosis of lower-grade gliomas (28). Alterations of metabolism-related lncRNA AP005264.1 expression were strongly correlated with poor prognosis in osteosarcoma (29). In addition, other lncRNAs in this study have not been reported in the relevant literature.
The TME is a complex and evolving system composed mainly of non-tumor cells, extracellular matrix (ECM), vascular system, and non-cellular components (30). The immune microenvironment, composed of multiple immune cells, is an important component of the TME and can serve as a predictor of tumor immunotherapy efficacy (31). The high- and low-DRLI subgroups exhibited significantly different immune cell infiltration and immune function scores. Interestingly, the scores of CD8+ T cells, Th cells, B cells, Tfh cells, and TIL cells were significantly higher in the low-DRLI group than in the high-DRLI group. CD8+ T lymphocytes can differentiate into effector CTLs upon stimulation with tumor-associated antigens. CTLs can participate in the tumor immune response by transferring from the peripheral immune organs to the tumor site through chemokines. Tfh cells enhance CD8+ T lymphocytes functions by secreting IL-21. Th cells can participate in both CTL cell activation and secretion of cytokines with antitumor immune effects. B cells can differentiate into plasma cells with the ability to secrete antitumor antibodies upon stimulation with tumor-associated antigens. TIL cells are infiltrating lymphocytes isolated from tumor tissues. Some of these cells are T cells targeting tumor-specific mutant antigens, which are immune cells capable of penetrating deep into the interior of tumor tissues to kill tumors. As innate immune cells, NK cells, macrophages and NKT cells did not observe significant differences between the two subgroups. In summary, the anti-tumor ability of the low-DRLI group was stronger than that of the high-DRLI group. The TME results showed that the stromal score and the immune score of the low-DRLI group were higher than those of the high-DRLI group, which indicated that the high-DRLI group samples had less infiltration of immune cells, a low content of stromal cells, and a high purity of tumors, which was in line with the results assessed by the ssGSEA algorithm. These findings suggest that the tumor immune microenvironment in patients with PAAD in the high-DRLI group is immunosuppressive, and the low-DRLI group may have a stronger anti-tumor immune response, which explains the difference in prognosis between the two groups.
Today, ICIs have emerged as a novel focus for cancer treatment (32). Compared with conventional therapies, immune checkpoint inhibition therapy has shown rapid and consistent efficacy in certain cancer patients, especially in patients with advanced metastatic cancer (33,34). Our study found differences in the distribution of immune checkpoint genes in the two subgroups, with most of the genes being more highly expressed in the low-DRLI subgroups. Patients in the low-DRLI group may have a better therapeutic response to immune checkpoint inhibition therapy.
The higher the tumor mutational load, the greater the production of neoantigens that can be recognized by immune cells, and the better the efficacy of receiving immune checkpoint inhibitor therapy is likely to be (35,36). This study found higher TMB values in the high-DRLI group, suggesting that immunotherapy may be more beneficial in this subgroup. In a subsequent subgroup survival analysis, it was shown that the DRLI score was a more accurate predictor of prognosis in patients with PAAD compared to TMB.
There are some limitations in this study. First, there was no external validation of our results with other datasets due to the lack of lncRNA expression profiles. Second, we explored the potential mechanisms of different prognoses between high- and low-risk subgroups by pure bioinformatics analysis. However, the results need to be verified by more funda-mental experiments.
Conclusions
The present study implicates that several DRlncRNAs (TM4SF1.AS1, AL031722.1, AP005264.1, AL358472.2, AC021087.1) may serve as independent prognostic biomarkers for pancreatic cancer. Our study also demonstrated that this lncRNAs are strongly correlated with the immune microenvironment, mutational burden, and response to immunotherapy in pancreatic cancer. This research may provide a new view for the clinical treatment of pancreatic cancer.
Acknowledgments
We would like to acknowledge the TCGA database for free data use.
Funding: None.
Footnote
Reporting Checklist: The authors have completed the TRIPOD reporting checklist. Available at https://tcr.amegroups.com/article/view/10.21037/tcr-23-1706/rc
Peer Review File: Available at https://tcr.amegroups.com/article/view/10.21037/tcr-23-1706/prf
Conflicts of Interest: All authors have completed the ICMJE uniform disclosure form (available at https://tcr.amegroups.com/article/view/10.21037/tcr-23-1706/coif). The authors have no conflicts of interest to declare.
Ethical Statement: The authors are accountable for all aspects of the work in ensuring that questions related to the accuracy or integrity of any part of the work are appropriately investigated and resolved. The study was conducted in accordance with the Declaration of Helsinki (as revised in 2013).
Open Access Statement: This is an Open Access article distributed in accordance with the Creative Commons Attribution-NonCommercial-NoDerivs 4.0 International License (CC BY-NC-ND 4.0), which permits the non-commercial replication and distribution of the article with the strict proviso that no changes or edits are made and the original work is properly cited (including links to both the formal publication through the relevant DOI and the license). See: https://creativecommons.org/licenses/by-nc-nd/4.0/.
References
- Siegel RL, Miller KD, Wagle NS, et al. Cancer statistics, 2023. CA Cancer J Clin 2023;73:17-48. [Crossref] [PubMed]
- Kleeff J, Korc M, Apte M, et al. Pancreatic cancer. Nat Rev Dis Primers 2016;2:16022. [Crossref] [PubMed]
- Rawla P, Sunkara T, Gaduputi V. Epidemiology of Pancreatic Cancer: Global Trends, Etiology and Risk Factors. World J Oncol 2019;10:10-27. [Crossref] [PubMed]
- Halbrook CJ, Lyssiotis CA, Pasca di Magliano M, et al. Pancreatic cancer: Advances and challenges. Cell 2023;186:1729-54. [Crossref] [PubMed]
- Koren E, Fuchs Y. Modes of Regulated Cell Death in Cancer. Cancer Discov 2021;11:245-65. [Crossref] [PubMed]
- Galluzzi L, Vitale I, Aaronson SA, et al. Molecular mechanisms of cell death: recommendations of the Nomenclature Committee on Cell Death 2018. Cell Death Differ 2018;25:486-541. [Crossref] [PubMed]
- Tang D, Kang R, Berghe TV, et al. The molecular machinery of regulated cell death. Cell Res 2019;29:347-64. [Crossref] [PubMed]
- Liu X, Olszewski K, Zhang Y, et al. Cystine transporter regulation of pentose phosphate pathway dependency and disulfide stress exposes a targetable metabolic vulnerability in cancer. Nat Cell Biol 2020;22:476-86. [Crossref] [PubMed]
- Zheng P, Zhou C, Ding Y, et al. Disulfidptosis: a new target for metabolic cancer therapy. J Exp Clin Cancer Res 2023;42:103. [Crossref] [PubMed]
- Li XM, Liu SP, Li Y, et al. Identification of disulfidptosis-related genes with immune infiltration in hepatocellular carcinoma. Heliyon 2023;9:e18436. [Crossref] [PubMed]
- Yang L, Zhang W, Yan Y. Identification and characterization of a novel molecular classification based on disulfidptosis-related genes to predict prognosis and immunotherapy efficacy in hepatocellular carcinoma. Aging (Albany NY) 2023;15:6135-51. [Crossref] [PubMed]
- Liu J, Peng WX, Mo YY, et al. MALAT1-mediated tumorigenesis. Front Biosci (Landmark Ed) 2017;22:66-80. [Crossref] [PubMed]
- Xing C, Sun SG, Yue ZQ, et al. Role of lncRNA LUCAT1 in cancer. Biomed Pharmacother 2021;134:111158. [Crossref] [PubMed]
- Su Y, Gu X, Zheng Q, et al. LncRNA PCGEM1 in Human Cancers: Functions, Mechanisms and Promising Clinical Utility. Front Oncol 2022;12:847745. [Crossref] [PubMed]
- Wang T, Guo K, Zhang D, et al. Disulfidptosis classification of hepatocellular carcinoma reveals correlation with clinical prognosis and immune profile. Int Immunopharmacol 2023;120:110368. [Crossref] [PubMed]
- Ternès N, Rotolo F, Michiels S. Empirical extensions of the lasso penalty to reduce the false discovery rate in high-dimensional Cox regression models. Stat Med 2016;35:2561-73. [Crossref] [PubMed]
- Balachandran VP, Gonen M, Smith JJ, et al. Nomograms in oncology: more than meets the eye. Lancet Oncol 2015;16:e173-80. [Crossref] [PubMed]
- Hänzelmann S, Castelo R, Guinney J. GSVA: gene set variation analysis for microarray and RNA-seq data. BMC Bioinformatics 2013;14:7. [Crossref] [PubMed]
- Yoshihara K, Shahmoradgoli M, Martínez E, et al. Inferring tumour purity and stromal and immune cell admixture from expression data. Nat Commun 2013;4:2612. [Crossref] [PubMed]
- Schmitt AM, Chang HY. Long Noncoding RNAs in Cancer Pathways. Cancer Cell 2016;29:452-63. [Crossref] [PubMed]
- Ghafouri-Fard S, Fathi M, Zhai T, et al. LncRNAs: Novel Biomarkers for Pancreatic Cancer. Biomolecules 2021;11:1665. [Crossref] [PubMed]
- Lei S, He Z, Chen T, et al. Long noncoding RNA 00976 promotes pancreatic cancer progression through OTUD7B by sponging miR-137 involving EGFR/MAPK pathway. J Exp Clin Cancer Res 2019;38:470. [Crossref] [PubMed]
- Zhou B, Guo W, Sun C, et al. Linc00462 promotes pancreatic cancer invasiveness through the miR-665/TGFBR1-TGFBR2/SMAD2/3 pathway. Cell Death Dis 2018;9:706. [Crossref] [PubMed]
- Pan S, Shen M, Zhou M, et al. Long noncoding RNA LINC01111 suppresses pancreatic cancer aggressiveness by regulating DUSP1 expression via microRNA-3924. Cell Death Dis 2019;10:883. [Crossref] [PubMed]
- Kitajima H, Maruyama R, Niinuma T, et al. TM4SF1-AS1 inhibits apoptosis by promoting stress granule formation in cancer cells. Cell Death Dis 2023;14:424. [Crossref] [PubMed]
- Zeng Z, Shi Z, Liu Y, et al. HIF-1α-activated TM4SF1-AS1 promotes the proliferation, migration, and invasion of hepatocellular carcinoma cells by enhancing TM4SF1 expression. Biochem Biophys Res Commun 2021;566:80-6. [Crossref] [PubMed]
- He C, Qi W, Wang Z. Effect and mechanism of downregulating the long-chain noncoding RNA TM4SF1-AS1 on the proliferation, apoptosis and invasion of gastric cancer cells. World J Surg Oncol 2021;19:226. [Crossref] [PubMed]
- Lin JZ, Lin N, Zhao WJ. Identification and validation of a six-lncRNA prognostic signature with its ceRNA networks and candidate drugs in lower-grade gliomas. Genomics 2020;112:2990-3002. [Crossref] [PubMed]
- Chen X, Ye Z, Lou P, et al. Comprehensive analysis of metabolism-related lncRNAs related to the progression and prognosis in osteosarcoma from TCGA. J Orthop Surg Res 2021;16:523. [Crossref] [PubMed]
- Anderson NM, Simon MC. The tumor microenvironment. Curr Biol 2020;30:R921-5. [Crossref] [PubMed]
- Zhang Z, Qiu X, Yan Y, et al. Evaluation of Ferroptosis-related Gene AKR1C1 as a Novel Biomarker Associated with the Immune Microenvironment and Prognosis in Breast Cancer. Int J Gen Med 2021;14:6189-200. [Crossref] [PubMed]
- Topalian SL, Wolchok JD, Chan TA, et al. Immunotherapy: The path to win the war on cancer? Cell 2015;161:185-6. [Crossref] [PubMed]
- Flynn MJ, Sayed AA, Sharma R, et al. Challenges and Opportunities in the Clinical Development of Immune Checkpoint Inhibitors for Hepatocellular Carcinoma. Hepatology 2019;69:2258-70. [Crossref] [PubMed]
- Xu F, Jin T, Zhu Y, et al. Immune checkpoint therapy in liver cancer. J Exp Clin Cancer Res 2018;37:110. [Crossref] [PubMed]
- Samstein RM, Lee CH, Shoushtari AN, et al. Tumor mutational load predicts survival after immunotherapy across multiple cancer types. Nat Genet 2019;51:202-6. [Crossref] [PubMed]
- Yarchoan M, Hopkins A, Jaffee EM. Tumor Mutational Burden and Response Rate to PD-1 Inhibition. N Engl J Med 2017;377:2500-1. [Crossref] [PubMed]